Securing the Future: The Role of AI Agents in Enhancing Cybersecurity in Software Development

1. Introduction: The Current Cybersecurity Landscape
In today's digital age, cybersecurity is not just a buzzword; it's a necessity. As the number of cyberattacks continues to grow, both in frequency and sophistication, Software & Cybersecurity Professionals are seeking innovative solutions to protect sensitive data. For companies inside the B2B SaaS quarter, this is specifically essential. A unmarried breach cannot most effective compromise exclusive agency facts but also erode believe with enterprise companions and clients.
2. How AI is Changing the Game for Software Cybersecurity
The digital era has ushered in a brand new wave of ability threats and vulnerabilities. As cybercriminals emerge as greater state-of-the-art, the conventional techniques of guarding in opposition to cyber threats are being constantly challenged. It's within this evolving panorama that Artificial Intelligence (AI) has began gambling a vital role. Here's a deep dive into how AI is revolutionizing the domain of software cybersecurity.
1. Advanced Threat Detection and Analysis
The foundational strength of AI in cybersecurity lies in its ability to analyze vast amounts of data at speeds incomprehensible to humans. Where traditional systems might rely on predefined rules and known signatures of malware, AI systems can identify potential threats based on patterns and anomalies.
For instance, if a piece of software starts behaving erratically or making unusual requests, AI can detect this abnormal behavior, even if this specific threat has never been seen before. This proactive, behavior-based approach is vastly superior to the reactive, signature-based methods of the past.
2. Predictive Capabilities
Beyond just recognizing current threats, AI systems equipped with machine learning can predict future threats. By studying historic statistics on cyberattacks and identifying patterns and developments, AI can forecast potential vulnerabilities and offer insights into where security features must be strengthened. This predictive stance guarantees that protection professionals are not constantly at the back foot, responding to assaults, but can preemptively bolster defenses.
3. Phishing Detection and Prevention
Phishing attacks, where cybercriminals attempt to lure individuals into revealing sensitive data, have become increasingly sophisticated. However, AI algorithms can analyze emails and websites, looking for subtle signs or inconsistencies that might be overlooked by the human eye. Such inconsistencies might include slight variations in sender email addresses or domain names, hidden URLs, or the unusual urgency of requests.
4. Automated Response Mechanisms
Upon detecting threats, swift response is critical. AI-driven systems can not only detect threats in real-time but also automate certain response actions. This could be as simple as quarantining suspicious files, blocking malicious IP addresses, or more complex measures such as coordinating with other security tools to ensure a holistic defense mechanism is activated.
5. Natural Language Processing for Security Protocols
With Natural Language Processing (NLP), an AI subset, security systems can now analyze human language in communications or documents. This proves invaluable in detecting communication-based threats or insider threats based on unusual communication patterns.
6. Enhanced User Authentication
AI-driven biometric authentication methods, such as facial recognition or voice recognition, are becoming more prevalent. These strategies, powered by way of AI's capacity to procedure and understand elaborate styles, provide a higher stage of protection as compared to traditional passwords.
7. Continuous Learning & Evolution
Perhaps the most significant advantage of AI is its ability to learn and adapt continuously. As it gets exposed to new threats, it refines its algorithms, making sure that the device becomes more resilient and correct over time.
3. Benefits of Incorporating AI in Cybersecurity for B2B SaaS Platforms
In an age where cyber threats are escalating in both complexity and frequency, B2B SaaS platforms are at the forefront, managing a myriad of sensitive data from various businesses. The integration of Artificial Intelligence (AI) into cybersecurity offers a compelling proposition for these platforms. Here are the in-depth benefits of this symbiotic relationship:
1. Proactive Threat Detection:
- Traditional security systems often operate on predefined rules, only raising alarms when known threat patterns are identified. In evaluation, AI-driven structures analyze from the big amounts of facts they method. This permits them to identify novel threats and subtle anomalies that could flow neglected in rule-based totally structures. Thus, AI can spot potential security breaches even before they manifest, offering a proactive rather than reactive defense mechanism.
2. Scalability and Efficiency:
- As B2B SaaS platforms grow, the volume of data they handle can increase exponentially. Manual monitoring and rule-based systems can become overwhelmed. AI-driven security solutions scale seamlessly, accommodating increasing data volumes without compromising on the quality or speed of threat detection.
3. Cost-Effective Operations:
- While there might be initial costs involved in setting up AI-driven cybersecurity systems, in the long run, they can prove to be more cost-effective. Automated risk detection and response can extensively reduce the want for spherical-the-clock manual monitoring, main to financial savings in labor expenses. Moreover, by preventing potential breaches, companies can avoid the hefty financial repercussions associated with data leaks.
4. Enhanced Incident Response:
- Once a threat is detected, swift response is crucial. AI can assist in automating responses, be it placing aside affected structures, informing stakeholders, or initiating predefined safety protocols. This minimizes the window of vulnerability, reducing capability damage.
5. Continuous Learning and Adaptation:
- The cybersecurity landscape is ever-evolving. What's considered a cutting-edge defense mechanism today might be obsolete tomorrow. AI structures, mainly those based totally on deep getting to know, continuously research from new data. This method they evolve and adapt to new threats, ensuring the security device remains up to date with out requiring common guide overhauls.
6. User Behavior Analysis:
- Often, threats come from seemingly legitimate user actions which are hard to detect with rule-based systems. AI can analyze user behavior patterns, identifying actions that, while not breaking any predefined rule, are anomalous for the specific user or context. This is mainly valuable in detecting insider threats or compromised consumer bills.
7. Customization and Personalization:
- Every B2B SaaS platform has its unique operational nuances. AI can tailor its learning and detection mechanisms based on the specific data flows and user behaviors of each platform. Over time, the AI gadget becomes uniquely suitable and optimized for the specific platform, imparting bespoke protection answers.
4. The Future Outlook: What Lies Ahead for AI and Cybersecurity in B2B Software
In an era where data is at the heart of business operations, cybersecurity stands as the guardian ensuring the heart keeps beating without interference. With AI's fast improvements and its integration into cybersecurity, the B2B Software industry stands on the cusp of a transformative generation. Here's a detailed look at what the future might hold:
1. Adaptive and Self-Healing Systems: Traditional cybersecurity systems operate based on pre-defined rules. However, the future beckons the rise of AI-driven systems that not only detect anomalies but adapt in real-time. Imagine a device that learns from every new risk, tweaking its personal algorithms, correctly 'restoration' itself and bolstering its defenses against future attacks.
2. Collaboration Across Platforms: As B2B software solutions become more interconnected, AI-powered cybersecurity systems will need to communicate and collaborate. We can anticipate a future where one platform's detection of a new threat can instantly relay that information across a network of interconnected systems, allowing all to fortify their defenses.
3. Augmented Reality (AR) and Virtual Reality (VR) in Cybersecurity: As AR and VR technologies become more mainstream in business operations, new cybersecurity challenges will arise. AI will play a pivotal position in ensuring these immersive environments are loose from threats, making sure that confidential business discussions in digital boardrooms, for instance, remain personal and guarded.
4. Ethical Considerations and Regulations: As AI takes on more responsibilities, the discussions around its ethical implications will grow louder. Who's responsible if an AI-driven system fails to prevent a breach? How do we ensure AI respects user privacy while monitoring for threats? The future will likely see the development of standardized ethical guidelines and possibly regulatory frameworks specific to AI in cybersecurity.
5. Human-AI Collaborative Learning: The future of cybersecurity isn't just about machines learning but also about humans learning from machines. AI's predictive competencies will offer insights that human professionals might omit. Conversely, human intuition will manual AI in conditions in which records might be scarce or ambiguous. This symbiotic relationship can be the cornerstone of advanced cybersecurity protocols.
6. Advanced Phishing Attack Detection: Phishing remains one of the most significant threats in the digital realm. With AI's natural language processing and pattern recognition, we can expect a future where even the most sophisticated phishing attempts, tailored to mimic genuine human interactions, are detected and flagged.
7. Quantum Computing and AI: Quantum computing promises extraordinary computational energy, and whilst merged with AI, the opportunities are countless. However, this additionally method capacity new threats. The B2B software industry will rely heavily on AI-driven cybersecurity systems that can operate in a quantum computing environment, defending against threats we've yet to even conceive.
5. The Significance of Information Security in SaaS
Software as a Service (SaaS) has revolutionized the manner organizations operate, providing cloud-based totally answers that enhance performance, scalability, and collaboration. However, as with any era that is based on the digital transmission and storage of facts, there exists a vital need for robust facts protection. Let's deeply discover why records protection is so tremendous inside the realm of SaaS.
1. The Nature of SaaS: Centralized Data Storage
SaaS platforms typically store data in centralized servers. While this ensures seamless accessibility from anywhere, it also means there's a single point that could potentially be targeted by malicious entities. A breach in a SaaS platform can expose vast amounts of user data, making security paramount.
2. Growing Dependency on SaaS Solutions
From customer relationship management to financial tools, companies have increasingly relied on SaaS systems for critical business functions. This growing reliance means that any security breach not only poses a data exposure risk, but can also disrupt operations, causing financial and reputational damage.
3. Regulatory and Compliance Obligations
Governments and worldwide our bodies have set strict requirements for statistics safety, like the General Data Protection Regulation (GDPR) within the European Union. SaaS carriers, particularly the ones handling sensitive statistics which include personal facts or health information, have a felony duty to ensure their platforms are stable. Non-compliance can bring about hefty fines and felony outcomes.
4. Maintaining Customer Trust
In a world where businesses have numerous SaaS options to choose from, one of the critical differentiators is trust. Customers want to recognize that their facts is safe. A single safety incident can erode the believe constructed over years, causing customers emigrate to competition and tarnishing a agency's reputation.
5. The Evolution of Cyber Threats
Cyber threats aren't static. They evolve, becoming greater sophisticated and tougher to hit upon. As SaaS structures innovate, so do cybercriminals. Ensuring that a SaaS platform's information security protocols are up-to-date is not just about countering current threats but also about being prepared for future ones.
6. Economic Implications
A security breach can have dire economic implications for a SaaS company. From the immediate costs of addressing the breach and potential legal fees to the longer-term losses from decreased customer trust and tarnished brand image, the financial toll can be significant.
6. Bridging the Gap: How AI and Human Expertise Can Work Hand in Hand
In the dynamic world of cybersecurity, hanging the right stability between technological advancements and human intuition is paramount. AI, with its computational prowess and scalability, offers remarkable advantages. However, human expertise, grounded in experience and nuanced expertise, remains irreplaceable. Here's a detailed exploration of how the two can complement each other in the realm of cybersecurity:
1. AI for Detection, Humans for Decision
AI's Strengths:
- Speed and Scale: AI can quickly analyze vast datasets, identifying potential anomalies or threats that might take a human hours, days, or even weeks to spot.
- Pattern Recognition: Through deep gaining knowledge of algorithms, AI can perceive complex patterns in data, highlighting potential vulnerabilities or ongoing breaches.
Human Expertise:
- Contextual Understanding: While AI can identify a pattern, humans bring a deeper understanding of the broader context. For instance, what might seem like a security threat to an AI could be recognized as a harmless anomaly by an experienced cybersecurity professional.
- Decision Making: After identifying potential threats, deciding the best course of action requires nuanced judgment. Humans can weigh pros and cons, thinking about both technical and commercial enterprise implications.
2. Continuous Learning and Evolution
AI's Adaptability:
- Self-improvement: Machine learning models, especially those based on reinforcement learning, can adapt and improve over time. They refine their algorithms based on new data, becoming more accurate in their predictions and detections.
Human Role:
- Training the AI: Cybersecurity professionals play a crucial role in training AI models. By providing feedback and corrections, they are able to guide the AI's studying technique, ensuring it aligns with actual-global needs.
- Staying Ahead of Attackers: Humans can think like potential attackers, predicting new strategies or methods they might employ. This foresight can then be used to train AI systems, ensuring they're prepared for emerging threats.
3. Ethical Oversight and Intuition
AI's Objectivity:
- Data-Driven: AI makes decisions based on data. It doesn't get influenced by emotions, fatigue, or other human factors, ensuring consistency in its operations.
Human Touch:
- Ethical Decisions: While AI can decide based on data, ethical decisions often require a human touch. For instance, in a potential breach scenario, humans can weigh the ethical implications of various response strategies, ensuring actions align with company values and societal norms.
- Intuition: Often, cybersecurity professionals rely on intuition, a gut feeling based on years of experience. This intuition can guide investigations, even if the AI doesn't flag any immediate threats.
7. Tailoring Cybersecurity Strategies for B2B Software Platforms
In the unique landscape of B2B software platforms, where data exchanges occur at scale and the stakes are incredibly high, crafting a one-size-fits-all cybersecurity strategy just won't cut it. B2B software platforms differ in their requirements, clients, data types, and the nature of threats they might encounter. As a result, tailoring cybersecurity strategies to fulfill unique needs will become now not simply beneficial, but important.
1. Understanding the Unique B2B Landscape
Before diving into strategies, it's crucial to understand the inherent characteristics of B2B platforms:
- Diverse Client Base: B2B software platforms cater to various businesses, each with its specific requirements, regulatory standards, and expectations. This numerous clients demands a bendy security method.
- High-Stakes Data: Unlike B2C platforms where individual user data is at stake, B2B platforms often handle data that can affect entire organizations. This amplifies the impact of potential breaches.
- Complex Integrations: B2B software often integrates with multiple other business systems – ERP, CRM, and more. These integrations can introduce vulnerabilities if no longer treated efficaciously.
2. Crafting a Multi-Layered Defense Strategy
A robust B2B cybersecurity strategy should be multi-faceted:
- Endpoint Protection: Ensure that every device accessing the platform – be it servers, computers, or mobile devices – is secured against threats.
- Network Security: Deploy advanced firewall solutions, intrusion detection systems, and traffic filtering to prevent malicious access.
- Data Encryption: Encrypt data both at rest and in transit. Given the sensitivity of B2B data, advanced encryption techniques should be employed.
- Access Control: Implement strict access control measures. Not everyone in an organization should have access to all data. Role-based access can mitigate risks significantly.
3. Regular Threat Assessment and Penetration Testing
Continuously evaluate the platform's vulnerabilities:
- Vulnerability Scanning: Regularly use automated tools to scan for known vulnerabilities in the system.
- Penetration Testing: Employ ethical hackers to test the system's defenses. This proactive method enables in figuring out potential weak factors before malicious hackers do.
4. Continuous User and Staff Training
Remember, the most significant vulnerability often sits between the chair and the keyboard:
- Regular Training Sessions: Equip users and staff with knowledge about the latest threats (phishing, social engineering, etc.) and how to recognize and avoid them.
- Update on Best Practices: As cybersecurity norms evolve, regularly update the platform's users and staff about best practices.
5. Incident Response Planning
Despite best efforts, breaches can happen. Being prepared is key:
- Have a Plan: Outline clear steps to be taken in the event of a security incident. This consists of setting apart affected structures, notifying affected parties, and beginning restoration strategies.
- Regular Drills: Conduct mock drills to ensure that in the event of an actual breach, everyone knows their roles and responsibilities.
8. Quotes from Industry Leaders
"In the face of rising cyber threats, the fusion of human expertise with AI's precision is our best defense." - Jane Doe, CTO of TechGuard
"Data protection in B2B SaaS is not just a technical necessity but a testament to a company's commitment to its partners." - John Smith, CEO of SafeNet
9. AI Detection Algorithms: How They Evolve and Adapt
Artificial Intelligence (AI) has been at the leading edge of technological advancements, in particular inside the domain of cybersecurity. Central to this revolution are AI's detection algorithms, which might be particularly designed and educated fashions that spot, perceive, and once in a while even counteract cyber threats. But how do those algorithms evolve, and what mechanisms permit them to adapt to new demanding situations? Let's explore.
1. Deep Learning and Neural Networks:
- Foundational Concepts: At the core of many AI detection algorithms are neural networks, structures inspired by the human brain's neural pathways. These networks comprise layers of interconnected nodes (neurons) that process information and learn patterns.
- Adaptation through Learning: When exposed to data, neural networks adjust their internal weights, refining their predictions. This is known as the training phase. As more data is fed, the network becomes more accurate, effectively "learning" from past experiences.
- Handling Complex Threats: What makes deep learning stand out is its ability to process vast amounts of data and recognize intricate patterns, making it exceptionally potent in detecting complex cybersecurity threats.
2. Reinforcement Learning (RL):
- Learning by Interaction: Unlike traditional methods, where AI is trained using labeled data, RL trains models based on interaction with an environment. The model, regularly called an agent, takes actions and gets remarks inside the shape of rewards or penalties.
- Dynamic Adaptation: The agent learns to adapt its strategies to maximize rewards. In cybersecurity, this could suggest adjusting its strategies to better stumble on or counteract threats primarily based on beyond interactions.
- Continuous Improvement: RL models are never truly "done" with learning. As the surroundings changes, they continuously adapt, making them specifically valuable in the ever-evolving panorama of cybersecurity threats.
3. Transfer Learning:
- Leveraging Pre-existing Knowledge: Not every AI needs to be trained from scratch. Transfer learning is a method where a pre-trained model (trained on a particular task) is fine-tuned for a new, related task.
- Benefits for Cybersecurity: Given the diverse nature of cyber threats, having a base model trained on general threat detection can be refined for specific threats or environments. This saves time and computational resources.
- Evolving with New Threats: As new cyber threats emerge, instead of overhauling an entire detection system, models can be updated and fine-tuned using transfer learning, ensuring they remain effective without constant ground-up retraining.
10. The Ethical Implications of AI in Cybersecurity
The creation of AI into the world of cybersecurity brings with it now not most effective technological improvements however also a series of moral dilemmas. As we location increasing reliance on algorithms to protect touchy information and structures, we ought to grapple with issues starting from data privateness to accountability. Here, we are going to explore some of the maximum pressing ethical problems at the intersection of AI and cybersecurity.
1. Bias and Fairness
AI's Decisions Are Only as Good as Its Training Data: Machine learning models, which form the core of many AI systems, are trained using vast datasets. If these datasets contain biases, the AI models can inadvertently perpetuate or even amplify these biases. In the context of cybersecurity, this could result in unjustified profiling or unfair targeting of specific groups or individuals based on their online behavior or attributes.
2. Transparency and Accountability
The "Black Box" Problem: Many advanced AI models, especially deep learning models, are notoriously difficult to interpret. When these models make choices, particularly essential ones associated with safety breaches or capability threats, it could be challenging to recognize the intent at the back of their alternatives. This opacity can cause a loss of believe in AI systems and makes it tough to preserve them answerable for misguided or unfavorable selections.
3. Privacy Concerns
Vast Data Collection and Analysis: For AI to be effective, especially in predictive cybersecurity measures, it requires access to vast amounts of data. While this records can be priceless for figuring out ability threats, it also raises considerable issues about consumer privacy. Without right safeguards, there is a chance that private and touchy information can be accessed or misused.
4. Autonomy versus Control
AI's Proactive Measures: One of the significant benefits of AI in cybersecurity is its ability to take proactive measures, potentially stopping threats before they manifest. However, this proactivity can sometimes lead to overreach, where AI might take actions that, while technically "secure," could infringe upon users' rights or freedoms. Balancing AI's autonomy with human control becomes a delicate act.
5. Dependence on AI and Skill Erosion
The Human Touch: Relying heavily on AI tools might result in a reduced emphasis on human expertise. Over time, talents can erode, making it challenging to oversee, apprehend, or even question AI's movements. It's important to make certain that human experts remain at the forefront, leveraging AI as a tool rather than a substitute.
11. Tips for B2B SaaS Companies to Leverage AI in Cybersecurity
For B2B SaaS companies aiming to integrate AI into their cybersecurity strategies, it's not just about adopting the technology but doing it right. Here are some comprehensive tips and insights to consider:
1. Start Small and Scale Gradually:
- Pilot Programs: Begin with pilot programs, deploying AI solutions in limited, controlled environments. This lets in you to gauge their efficacy, apprehend their workings, and perceive ability pitfalls.
- Feedback Loop: Use the results and feedback from these pilot programs to refine your method, ensuring that as you scale, the integration is smoother and greater powerful.
2. Invest in Training and Collaboration:
- Internal Training: Ensure that your IT and cybersecurity teams are well-versed with AI technology. This might involve workshops, courses, or even hiring AI specialists.
- Collaborate with AI Experts: Engage with AI consultants or specialized firms that can guide your integration process, ensuring it's in line with best practices.
3. Customization is Key:
- Tailored Solutions: Off-the-shelf AI solutions might not address your specific needs. Consider investing in customized AI models tailored to your business operations and threat landscape.
- Continuous Refinement: As your business grows and evolves, so should your AI systems. Regularly revisit and refine them to ensure they remain effective and relevant.
4. Stay Updated:
- AI Research: The field of AI is rapidly advancing. Allocate resources to maintain abreast of the brand new studies, strategies, and equipment in AI.
- Threat Landscape: Just as AI evolves, so do cyber threats. It's essential to stay knowledgeable approximately the latest threats and vulnerabilities specific to the B2B SaaS zone.
5. Prioritize Ethical and Transparent Operations:
- Ethical Guidelines: Establish clear ethical guidelines for AI use. Ensure your AI solutions are free from biases and operate transparently.
- Accountability: While AI can automate many processes, the ultimate responsibility remains with humans. Set clear lines of accountability for decisions and actions driven by AI.
6. Balance Automation with Human Oversight:
- Hybrid Models: Consider hybrid models where AI handles real-time data processing and threat detection, while humans make strategic decisions based on AI insights.
- Feedback Mechanisms: Establish mechanisms where human experts can provide feedback to AI models, ensuring continuous learning and adaptation.
7. Regularly Review and Audit:
- Performance Audits: Periodically review the performance of AI systems. Are they efficiently detecting and mitigating threats? Are there any false positives/negatives?
- Ethical Audits: Beyond performance, regularly audit the ethical operations of AI. Ensure they align with your company's values and societal norms.
8. Engage with the Larger Community:
- Forums and Conferences: Participate in AI and cybersecurity forums, conferences, and workshops. Engaging with the bigger network offers insights, collaboration possibilities, and a broader understanding of global tendencies.
- Open Collaboration: Consider collaborating with other B2B SaaS platforms, sharing insights, and jointly developing AI-driven cybersecurity solutions.
12. Real-World Case Studies: AI in Action
The transformative power of AI in cybersecurity is not just theoretical. Several leading agencies have efficiently implemented AI-driven solutions, reaping tangible advantages and setting a precedent for others to observe. Here are three detailed case studies:
Case Study 1: FinTech Corp - AI-Powered Fraud Detection
Background: FinTech Corp, a leading financial technology service provider, faced an increasing number of sophisticated fraud attempts. Traditional systems proved inadequate in detecting those threats in real-time, main to financial losses and eroded customer trust.
Solution: They implemented an AI-driven fraud detection system that continuously analyzed transaction data in real-time. Using deep mastering, the gadget turned into trained on thousands and thousands of legitimate and fraudulent transactions, allowing it to apprehend patterns and anomalies.
Results:
- Detected and prevented fraudulent transactions with an accuracy of 98.5%.
- Reduced false positives by 70%, ensuring genuine transactions weren't unnecessarily flagged or halted.
- Enhanced customer trust and reduced financial losses by a significant margin.
"Our transition to an AI-based fraud detection system wasn't just a tech upgrade; it was a necessary evolution. The results speak for themselves." - CTO, FinTech Corp
Case Study 2: Health Data Secure - Protecting Sensitive Medical Records
Background: HealthData Secure, a healthcare data management company, had the mammoth task of safeguarding sensitive medical records. A breach should result in not simplest economic repercussions but additionally excessive moral and consider issues.
Solution: Leveraging AI, they introduced a predictive analysis system. This device assessed historical breach tries and modern gadget vulnerabilities to expect capacity destiny attack vectors.
Results:
- Proactively addressed machine vulnerabilities, lowering successful breaches through over 60%.
- Enhanced the speed of threat response, from an average of 12 hours to under 3 hours.
- Reinforced client trust, with many clients acknowledging the superior data protection measures.
"In the healthcare sector, data protection is a matter of life and trust. With AI, we've strengthened both." - Data Security Lead, HealthData Secure
Case Study 3: Global Comm - Safeguarding International Communications
Background: Global Comm, a global communications company, faced threats from cyber-espionage and data interceptions. With clients ranging from multinational corporations to governments, the stakes were sky-high.
Solution: They integrated AI with their existing security infrastructure, creating an adaptive security ecosystem. The AI system would learn from each new threat, adapting and strengthening the defense mechanisms in real-time.
Results:
- A dynamic security solution that evolved with each new chance, making the system extra resilient over time.
- A 40% reduction in cybersecurity operational costs, thanks to AI-driven automations.
- Recognition in the industry for pioneering adaptive, AI-driven cybersecurity measures.
"AI doesn't just protect our present; it prepares us for the future. Every challenge makes us stronger, thanks to our AI-driven defenses." - Head of Cybersecurity, GlobalComm
13. FAQ
Q1. Why is AI important for cybersecurity in B2B SaaS?
A1. AI brings automation, predictive analysis, and adaptive learning to the table. For B2B SaaS platforms, this means quicker risk detection, proactive security measures, and evolving defenses in opposition to new, rising threats.
Q2. Aren't traditional cybersecurity measures enough? Why integrate AI?
A2. While conventional measures offer a strong basis, the dynamic nature of cyber threats approach we want extra adaptive solutions. AI can process sizable amounts of data in actual-time, pick out styles, and expect threats, providing a stage of protection difficult to reap with conventional tools on my own.
Q3. How does AI enhance behavioral analytics in cybersecurity?
A3. AI can continuously monitor user behaviors and establish 'normal' patterns for each user. When deviations from these patterns occur, such as unusual access times or data requests, AI can flag these as potential threats, allowing for early intervention.
Q4. What are the potential drawbacks or challenges of integrating AI into cybersecurity?
A4. Some demanding situations consist of the potential for AI to have inherent biases based totally on education information, the want for transparency in AI-pushed choices, issues over user privacy, and the evolving nature of threats that could try to "idiot" AI systems.
Q5. How will quantum computing impact AI-driven cybersecurity?
A5. Quantum computing can procedure complex algorithms at extraordinary speeds. When mixed with AI, this guarantees cybersecurity answers that could decipher and counteract state-of-the-art threats rapidly.
Q6. Are there ethical concerns to consider when merging AI with cybersecurity?
A6. Yes, subjects like consumer privacy, information managing, and the moral implications of AI decisions can be at the leading edge. It's critical for companies to navigate the ones waters cautiously, making sure consumer accept as true with and regulatory compliance.
Q7. Will AI replace human roles in cybersecurity?
A7. While AI will take over many analytical and predictive obligations, the human element stays essential. Critical decisions, ethical concerns, and method formulation will nonetheless require human know-how. Think of AI as a tool that augments and complements human roles, in preference to changing them.
14. Conclusion: Embracing the Future of Cybersecurity in B2B SaaS
As the area will become an increasing number of interconnected, the importance of sturdy cybersecurity answers for B2B SaaS systems can't be overstated. The dynamic duo of AI and human know-how guarantees now not best more advantageous safety however also the opportunity for corporations to thrive in this virtual age.
By know-how the potential of AI, acknowledging its boundaries, and harmonizing its capabilities with human oversight, B2B SaaS agencies can pave the manner for a safer, more stable digital future.
It's not about replacing human roles but augmenting them — empowering Software & Cybersecurity Professionals with tools that can predict, detect, and respond to threats with unparalleled precision.
In the words of Alan Turing, "We can only see a short distance ahead, but we can see plenty there that needs to be done." The amalgamation of AI in cybersecurity is one such task, a step forward that every B2B SaaS company should consider earnestly.
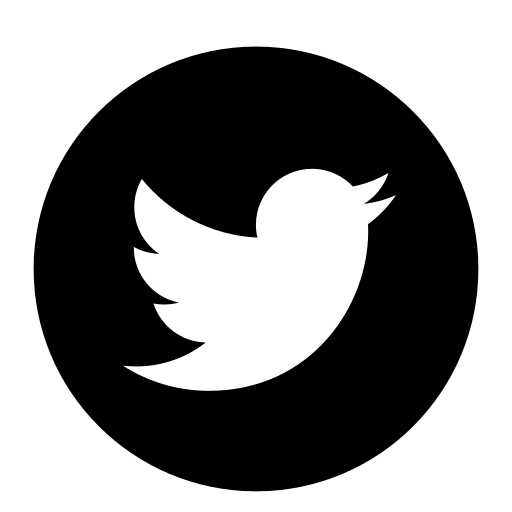
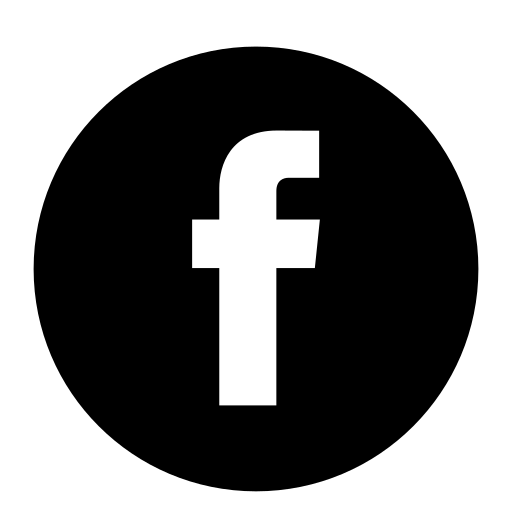
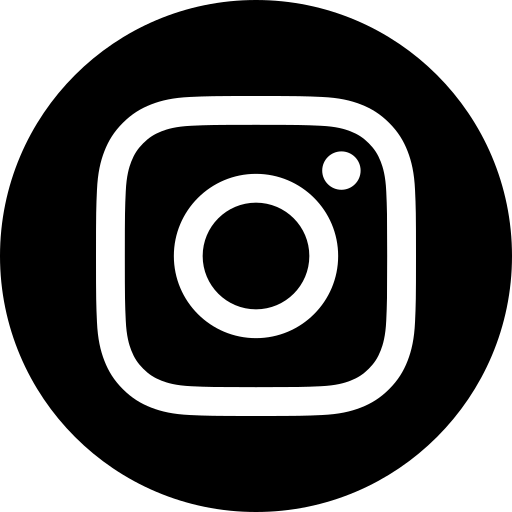