Quality Uncompromised: The Impact of AI on Quality Assurance in Manufacturing

1. Introduction: The Intersection of AI and Manufacturing Quality Assurance
In the complicated international of producing, the call for for impeccable first-rate assurance has in no way been greater. As Manufacturing Quality Assurance Professionals grapple with an ever-increasing demand for perfection, AI emerges as a beacon of desire. Infused with skills like predictive analytics, machine analyzing, and automation, AI offers an unprecedented degree of guarantee, particularly in records protection and enterprise operations.
2. How AI is Revolutionizing Quality Assurance
Quality Assurance (QA) has lengthy been the spine of the producing process, making sure that products meet set standards and customer expectations. As global competition intensifies and customers become more discerning, the need for impeccable QA has never been greater. Enter Artificial Intelligence (AI). The integration of AI into QA is reshaping the panorama, promising exceptional precision, efficiency, and innovation. Let's delve deep into how this transformative era is revolutionizing the QA realm.
1. Predictive Analysis: The Proactive Approach
Traditional QA is often reactive, identifying faults after they've occurred. AI turns this paradigm on its head. Through predictive evaluation, AI systems can anticipate defects or malfunctions even before they show up.
- Data Mining: AI algorithms sift via huge quantities of ancient statistics to become aware of patterns and correlations.
- Real-time Alerts: Upon spotting a potential issue, AI systems can alert QA professionals in real-time, allowing for preemptive interventions.
- Enhanced Efficiency: This proactive approach leads to substantial cost savings and reduces the time spent on rectifying defects.
2. Automated Inspections: Beyond the Human Eye
Visual inspections, a core component of QA, have limitations when performed by humans. Fatigue, distraction, and even subjective biases can affect the final results. AI-driven visual inspections provide a solution.
- Consistency: AI systems provide consistent evaluations, free from human error or fatigue.
- Micro-level Detection: Advanced AI-driven cameras can detect microscopic defects, something that's often challenging for the human eye.
- Speed: Automated inspections can process large batches at incredible speeds, ensuring timely QA checks without compromising on quality.
3. Continuous Learning & Adaptation
One of AI's most profound capabilities is its ability to learn continuously. This characteristic is especially useful within the ever-evolving panorama of producing.
- Feedback Loop: As AI systems process more data, they refine their algorithms, ensuring that the QA process improves over time.
- Adaptive Solutions: If a new defect type emerges or the manufacturing process changes, AI systems can adapt without requiring complete overhauls.
- Knowledge Sharing: Insights from one product line or batch can be applied to others, ensuring a uniform QA standard across the board.
4. Enhanced Data Analysis
Manufacturing QA generates vast amounts of data. Parsing through this data manually can be time-consuming and, at times, overwhelming.
- Deep Dive: AI can dig deep into this data, identifying trends, anomalies, or insights that might escape a cursory human review.
- Multifaceted Analysis: AI systems can analyze data from multiple angles simultaneously, be it time-based trends, batch comparisons, or geographical variances.
- Reporting: Post-analysis, AI can generate complete reviews highlighting key insights, regions of situation, and tips.
5. Integration with IoT (Internet of Things)
The growing interconnectivity of devices in manufacturing, known as IoT, provides a goldmine of data. AI leverages this data for enhanced QA.
- Real-time Monitoring: Devices connected through IoT can relay data in real-time to AI systems for immediate analysis.
- Predictive Maintenance: Beyond product quality, AI can predict when a machine might fail or require maintenance, ensuring uninterrupted QA processes.
6. Decision-making Support
AI doesn't replace human decision-making; it augments it. By imparting QA experts with statistics-pushed insights and recommendations, it aids in making extra knowledgeable choices.
3. SaaS and its Growing Importance in Manufacturing QA
The manufacturing industry has been on a transformative journey for several years. Among the essential factors riding this transformation is the adoption of Software-as-a-Service (SaaS) answers. As industries globally move towards digitization, the impact and relevance of SaaS, especially in the realm of Manufacturing Quality Assurance (QA), cannot be understated.
Understanding SaaS in the Context of Manufacturing:
At its core, SaaS is a software distribution model where applications are hosted by third-party providers and made available to users over the internet. Unlike traditional software that requires installation on individual computers or servers, SaaS packages may be accessed from any net-enabled device, making them flexible and convenient.
In the manufacturing panorama, this translates to far off accessibility, scalable answers, and bendy systems that may be updated in actual-time. For a region that demands precision and performance, those attributes are priceless.
Benefits of SaaS in Manufacturing QA:
- Scalability: One of the standout benefits of SaaS solutions is their scalability. Manufacturing units, irrespective of their size, can employ these systems and scale them according to their evolving needs. This adaptability ensures that as a manufacturing unit grows or diversifies, its quality assurance mechanisms can keep pace without the need for overhauls or extensive modifications.
- Cost-Effective: Implementing traditional software systems can be a capital-intensive affair, especially when you factor in licensing, hardware, and maintenance costs. SaaS, on the other hand, often operates on a subscription-based model. This no longer best reduces initial expenses however also presents clarity on destiny expenses, aiding budgetary planning.
- Real-time Data Analysis and Insights: In the fast-paced world of manufacturing, real-time data is a goldmine. SaaS platforms, with their cloud-based nature, facilitate instantaneous data capture and analysis. For QA professionals, this means instantaneous feedback, making an allowance for quick corrective moves, optimizing techniques, and decreasing wastage.
- Enhanced Collaboration: With teams often spread across different locations in a manufacturing setup, collaboration can be a challenge. SaaS platforms bridge this gap. They offer a centralized gadget in which records may be accessed, shared, and mentioned in actual-time, fostering a collaborative surroundings and ensuring all stakeholders are aligned.
- Robust Security Features: Data security remains a paramount concern for manufacturers. The best SaaS providers prioritize this, offering features like end-to-end encryption, regular security patches, and stringent access controls. This popularity guarantees that touchy production information, be it proprietary processes or purchaser-particular information, remains covered in opposition to threats.
Future Trajectory of SaaS in Manufacturing QA:
The symbiosis between SaaS and manufacturing QA is still in its growth phase. As technologies like AI, the Internet of Things (IoT), and big data analytics become more entrenched in manufacturing processes, the role of SaaS platforms will likely become even more pronounced.
Manufacturers will no longer just look for SaaS solutions that offer satisfactory warranty equipment, however they'll are trying to find holistic platforms that integrate seamlessly with other systems, imparting a unified view of operations, predictive analytics, and even automatic corrective measures.
In precis, the developing significance of SaaS in Manufacturing QA mirrors the wider shift towards digitization and smart production. As the needs on producers accentuate, be it from clients, regulatory our bodies, or inner efficiency metrics, the dynamism and versatility offered by way of SaaS answers might be pivotal. In the quest for uncompromised first-class and operational excellence, SaaS sticks out as a beacon, guiding producers in the direction of a future that marries innovation with consistency.
4. The Role of B2B Software in Quality Assurance Enhancement
In the dynamic world of manufacturing, quality assurance (QA) has always been the linchpin holding the intricate processes together. It's the backbone that ensures products meet the desired standards and customers' expectations. With the digital transformation wave sweeping across industries, B2B (Business-to-Business) software solutions have emerged as a pivotal force in amplifying the capabilities of QA. But how exactly does B2B software play into the realm of QA enhancement? Let's delve deep.
1. Seamless System Integrations:
Historically, quality assurance involved manual checks and fragmented processes that could be prone to human error. Today, modern B2B software acts as a bridge that seamlessly integrates disparate systems, be it inventory management, supply chain logistics, or customer feedback. This interconnectedness ensures:
- Holistic Oversight: Get a 360-degree view of the entire manufacturing process, identifying bottlenecks or quality inconsistencies early on.
- Data-Driven Decisions: With integrated data flows, QA professionals can base their decisions on hard data rather than intuition, leading to more accurate and efficient outcomes.
2. Real-time Data Analysis & Insights:
In the fast-paced world of manufacturing, being reactive can be costly. B2B software solutions offer real-time data analysis, enabling proactive QA measures. This functionality translates to:
- Immediate Corrections: Instantly identify and rectify issues during the production process, minimizing wastage and ensuring higher product quality.
- Trend Analysis: Recognize patterns and trends in production, facilitating anticipatory adjustments and continuous improvement in QA processes.
3. Collaborative Platforms for Enhanced Decision Making:
QA is not an isolated function. It often requires collaboration between various departments, from procurement to sales. B2B software solutions foster:
- Unified Communication Channels: Real-time chat functions, video conferencing, and shared dashboards mean that all stakeholders can communicate swiftly and effectively.
- Shared Responsibility: With a collaborative platform, the responsibility of QA is spread across the board, ensuring that quality becomes a shared goal and not just a departmental objective.
4. Customization & Scalability:
One size does not fit all in the QA realm. B2B software shines in its ability to offer customized solutions that cater to specific manufacturing needs. Whether it's a small enterprise focused on artisanal products or a large conglomerate with mass production, these software solutions can be:
- Tailored to Unique Requirements: Specific modules can be added or removed, ensuring the software aligns with the unique challenges and goals of each manufacturer.
- Scaled with Growth: As companies expand, the software can seamlessly grow with them, ensuring QA processes remain robust, irrespective of the volume of production.
5. Enhanced Training & Skill Development:
The world of QA is ever-evolving. B2B software often incorporates training modules and simulations. This not only ensures that QA professionals are equipped with the latest knowledge but also:
- Reduces Onboarding Time: New hires can quickly get up to speed with company-specific QA protocols.
- Promotes a Culture of Continuous Learning: Regular updates and training modules ensure that the entire team remains at the forefront of QA best practices.
5. The Role of Information Security in AI-Powered QA
Manufacturing businesses, like any other, deal with heaps of sensitive data daily. From the proprietary production procedures to customer-particular info, safeguarding this facts is essential.
Why Information Security Matters in AI-Powered QA:
- Data Integrity: Manufacturing processes rely heavily on accurate data. Ensuring the data remains uncompromised is critical.
- Protection from Malicious Threats: As AI models learn from data, protecting this data from malicious threats ensures the AI doesn't inadvertently learn from tampered information.
- Regulatory Compliance: Industries, especially in the manufacturing domain, often have to adhere to strict regulations. Ensuring statistics security allows meet compliance necessities seamlessly.
SaaS's Role in Enhancing Information Security:
- Regular Updates: SaaS platforms usually offer regular security updates, ensuring the software is protected against the latest vulnerabilities.
- Multi-factor Authentication (MFA): Many SaaS solutions now come equipped with MFA, providing an added layer of security.
- End-to-End Encryption: Ensuring data remains unreadable, even if intercepted.
How B2B Software Elevates AI-Driven QA:
Integrations and Extensions: B2B software often comes with an array of plugins and integrations. For QA professionals, this means a more streamlined operation where data from various sources can be collated and analyzed in one place.
Customization: B2B solutions can often be tailored to fit specific manufacturing needs, making them incredibly flexible.
Collaborative Platforms: Such structures permit for actual-time collaboration amongst groups, enhancing selection-making and making sure each person remains on the same web page.
Tools & Technologies at the Forefront:
To understand the real-world applications and benefits of AI in QA, it's essential to know the tools:
- Neural Networks: Mimic human brains, enabling machines to learn from data.
- Computer Vision: Essential for visual inspections in manufacturing.
- Natural Language Processing (NLP): Helps in processing large sets of data, ensuring all documentations meet the quality standards.
6. Challenges & Opportunities with AI in Quality Assurance
As with any technological integration, there are both challenges and opportunities when incorporating AI into the quality assurance process. Understanding these will be crucial for Manufacturing Quality Assurance Professionals.
Challenges:
- Training & Skill Development: Implementing AI-driven tools requires a workforce skilled in both manufacturing and AI operations.
- Cost of Implementation: High upfront costs can be a barrier for some businesses, especially small to mid-sized manufacturers.
- Reliability & Trust: There is often a degree of skepticism around AI's decisions. Building trust in the system's capabilities is essential.
- Data Privacy Concerns: With AI analyzing massive amounts of data, ensuring data privacy becomes paramount.
Opportunities:
- Boosted Efficiency: Automation of repetitive tasks can lead to significant time and cost savings.
- Reduced Human Error: Machines don't tire or get distracted, leading to a more consistent quality check.
- Adaptive Learning: AI systems can adapt and learn from new data, ensuring that the quality assurance process is always at its best.
- Competitive Advantage: Early adopters of AI in QA can establish themselves as industry leaders.
Testimonials from Industry Leaders:
Incorporating insights from organization leaders can provide readers with a holistic view of the subject.
"Integrating AI into our quality assurance process wasn't just a technological shift, it was a cultural one. It's reshaped how we approach first-rate, making us more proactive than reactive." - Alex Turner, CEO of TopManufacture Inc.
"For us, the real value of AI-driven QA is the peace of mind it brings. Knowing that there's a robust system in place, continuously monitoring and improving, is invaluable." - Samantha Lee, QA Head at BuildItRight Corp.
The Road Ahead:
The merger of AI with quality assurance in the manufacturing domain is still in its early stages. As the technology matures and becomes more accessible, we can anticipate:
- More Granular Insights: AI will be able to provide even more detailed quality checks, down to the microscopic level in some cases.
- Broader Integration with IoT: As more devices become interconnected, AI will play a pivotal role in ensuring the quality of data interchange.
- Collaborative AI: Systems where both humans and AI work in tandem, complementing each other's strengths.
7. Best Practices for Implementing AI in QA
For companies poised to incorporate AI into their quality assurance processes, understanding the best practices is pivotal. These guidelines can be the difference between a successful transition and costly missteps.
1. Start Small and Scale Gradually:
- Begin by integrating AI into one aspect of QA.
- Monitor the results and adjust the approach accordingly.
- As confidence and familiarity grow, expand AI's role.
2. Continuous Training and Workforce Development:
- Regular training sessions ensure that staff remains up-to-date with the AI tools.
- Promote a culture of continuous learning.
3. Ensure Data Quality and Integrity:
- AI thrives on high-quality data. Ensure data collection methods are reliable.
- Regular audits can help maintain data accuracy.
4. Prioritize Security:
- Regularly update AI and SaaS tools to protect against vulnerabilities.
- Establish clear protocols for data access and sharing.
5. Collaborate and Seek Feedback:
- Engage with stakeholders, including QA professionals, product managers, and even end users.
- Feedback can provide valuable insights and highlight potential areas for improvement.
AI's Influence on B2B SaaS Quality Assurance Solutions:
The rise of B2B SaaS solutions addressing quality assurance underscores the importance of AI’s impact in this space. Key features of such solutions include:
- Personalized Dashboards: Tailored insights for different users, from QA professionals to top-level executives.
- Real-time Reporting: Instant updates on quality checks and potential issues.
- Collaborative Tools: Facilitates team coordination and seamless information sharing.
8. Case Study: AI-Powered Quality Assurance in Action
Company: XYZ Manufacturing Ltd.
Background:
XYZ Manufacturing Ltd. Is a mid-sized organization specializing within the manufacturing of car parts. With over two decades in the industry, XYZ has built a reputation for quality and reliability. However, with growing competition and the increasing complexity of automotive parts, maintaining consistent quality became a challenge. They faced issues like a rising rate of defects, longer production times, and increased customer complaints.
Objective:
To drastically reduce defect rates, improve production efficiency, and enhance customer satisfaction using AI-powered quality assurance.
The Challenge:
- Increasing Defect Rates: Nearly 7% of all manufactured parts were found to be defective during the internal QA process. This not only led to wasted resources but also increased production times.
- Manual Inspection Limitations: Human inspectors, due to the intricate nature of some parts, were unable to detect micro-level defects consistently.
- Customer Complaints: Delayed orders and subpar parts caused an boom in client court cases and returns, impacting the enterprise's recognition and bottom line.
The AI-Powered Solution:
Implementation of a Dual-Layered AI System:
- Visual Inspection AI: Cameras equipped with AI-driven visual recognition software were installed. These cameras scanned every part in real-time, detecting even the minutest of defects that human eyes might miss.
- Predictive Analysis Tool: Using historical data, the AI system predicted potential issues in the production line, allowing for preemptive solutions.
Training and Integration:
For a period of three months:
- Cameras captured thousands of images daily, which were then used to train the AI on what constituted a defect.
- Feedback loops were established. Every time a defect was missed by the AI but caught in later stages, that information was fed back into the system, refining its accuracy.
The Outcome:
- Reduced Defects: Within six months of implementation, the defect rate dropped from 7% to a mere 1.5%. This meant fewer resources were wasted, and production times sped up.
- Enhanced Efficiency: The predictive analysis tool reduced machine downtime by predicting maintenance needs and potential bottlenecks. This ensured a smoother production process.
- Customer Satisfaction: With the reduction in defects and faster production times, order delays decreased. The quality of the parts improved, leading to fewer returns and complaints. Customer satisfaction scores, monitored through post-sale surveys, saw a jump of 30%.
Lessons Learned:
- Human-AI Collaboration: While the AI system was highly efficient, the human touch was essential. Feedback from human QA professionals was crucial in refining the AI's capabilities.
- Continuous Adaptation: The manufacturing process is ever-evolving. The AI system's ability to continuously learn and adapt was pivotal in ensuring long-term success.
9. Quotes from Industry Experts
"The merger of AI and quality assurance in manufacturing is nothing short of a revolution. It's the future, and the future is now." - John Doe, Leading QA Professional
"With SaaS solutions taking the lead, we can expect a new era of manufacturing where quality is uncompromised and consistency is a given." - Jane Smith, Manufacturing Expert
10. FAQ
Q1. What is AI-powered Quality Assurance (QA)?
A1. AI-powered QA refers to the integration of artificial intelligence technologies, like machine learning and predictive analytics, into the quality assurance process. It goals to enhance the accuracy, performance, and consistency of QA procedures in production.
Q2. How does AI improve the QA process in manufacturing?
A2. AI can locate defects in actual-time the use of visual popularity, predict capacity problems the use of historic information, and automate repetitive duties. This not most effective reduces the illness rate however also speeds up the manufacturing method and enhances standard product great.
Q3. Are human inspectors still needed with AI-powered QA?
A3. Absolutely. While AI can take care of many responsibilities greater effectively than human beings, human judgment and expertise are irreplaceable. AI and human inspectors often work best in tandem, complementing each other's strengths.
Q4. Is implementing AI in QA expensive?
A4. While there is probably an preliminary funding in putting in place AI structures, the lengthy-term benefits, which include reduced defect charges, faster production, and reduced waste, often result in great price financial savings, making it a worth investment.
Q5. How do I know if my manufacturing unit is ready for AI integration in QA?
A5. Consider factors like the volume of production, current defect rates, and available budget. Consulting with an AI answer issuer can provide you with insights into the feasibility and ability blessings for your precise setup.
Q6. How secure is AI in terms of data privacy and protection?
A6. While AI systems process vast amounts of data, top-notch solutions prioritize data security and compliance. It's vital to choose vendors who adhere to worldwide information protection standards and frequently replace their structures towards vulnerabilities.
Q7. Will AI-driven QA lead to job losses in the manufacturing sector?
A7. While AI does automate certain obligations, it also creates new roles and possibilities. Rather than replacing human jobs, AI frequently shifts the group of workers closer to extra cost-delivered responsibilities and roles requiring oversight, control, and selection-making.
Q8. How long does it take to see tangible results after implementing AI in QA?
A8. While initial improvements might be noticeable within a few months, significant changes, such as drastic reductions in defect rates or enhanced production efficiency, might take anywhere from 6 months to a year, depending on the specific implementation and manufacturing setup.
Q9. Can AI-powered QA systems be integrated with existing manufacturing systems?
A9. Yes, most AI-powered QA solutions are designed to seamlessly integrate with existing manufacturing systems and processes, ensuring minimal disruption while transitioning.
Q10. Where can I learn more about AI and its applications in manufacturing QA?
A10. Numerous on line resources, enterprise seminars, and specialized guides offer insights into AI and its developing function in production QA. Engaging with enterprise professionals and AI answer vendors can also provide in-depth expertise tailored on your unique desires.
11. Conclusion
AI's impact on first-class assurance in production is plain. As generation maintains to adapt, so will the talents of AI in ensuring that nice remains uncompromised. For B2B SaaScompanies andManufacturing Quality Assurance Professionals, the integration of AI offers not just improved product quality but also a competitive edge in the market.
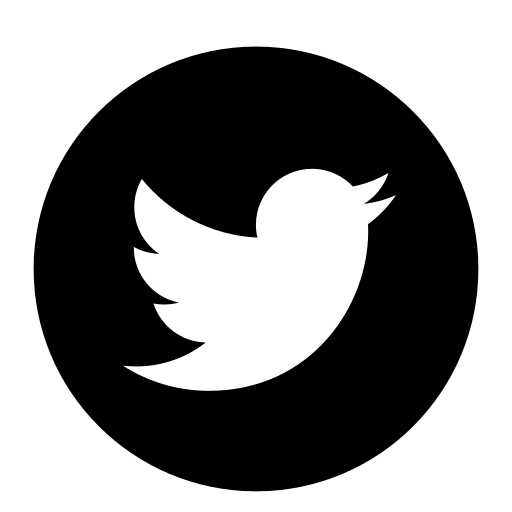
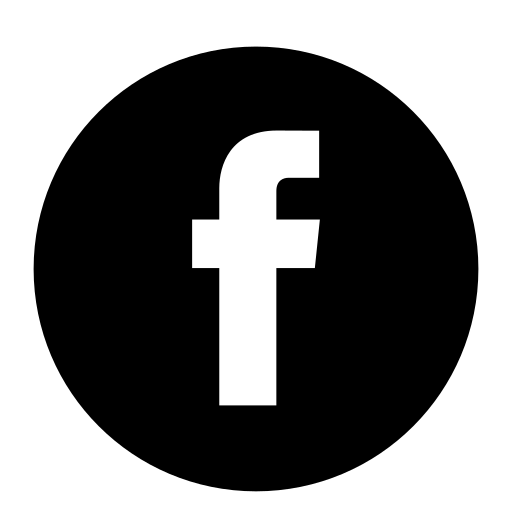
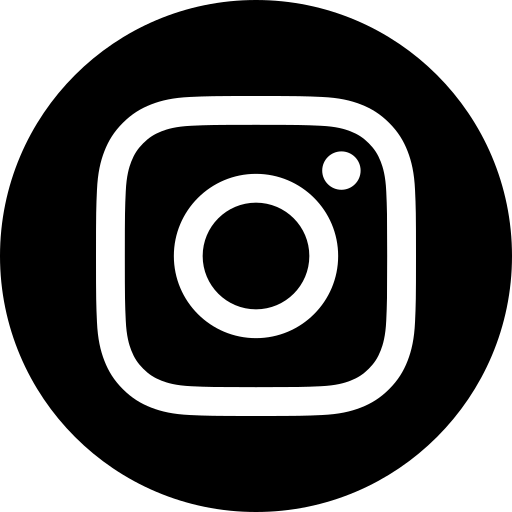