Product Development Propelled: How AI is Accelerating Innovation in the Tech Sector

1. Introduction to the AI Revolution in B2B SaaS
In the evolving landscape of the tech enterprise, Artificial Intelligence (AI) has emerged as a game-changer, revolutionizing every region from e-trade to healthcare. Particularly within the realm of B2B SaaS, AI's impact has been not anything quick of transformative. As corporations scramble to evolve and combine AI-driven gadget and methodologies, we discover ourselves on the cusp of an innovation explosion.
2. The Role of AI in Accelerating Product Development
The rapid evolution of generation has reshaped countless industries, and on the heart of this alteration is Artificial Intelligence (AI). AI's have an impact on on product development, mainly inside the B2B SaaS sector, can't be understated. To certainly respect its effect, we have to delve deeper into how AI quickens and refines product development techniques.
1. Automation and Efficiency
At a fundamental level, AI algorithms are designed to execute tasks without human intervention. For product developers, this automation is a sport-changer. Routine, time-eating duties including information entry, testing, or even trojan horse detection may be efficaciously handled by means of AI, releasing up developers to awareness on more strategic and innovative factors of the product. The result? Faster improvement cycles and merchandise that attain the market more speedy than ever earlier than.
2. Data-Driven Insights and Decision-Making
Today's virtual merchandise generate considerable quantities of facts, from consumer conduct to gadget performance metrics. Historically, making experience of this data become a huge task. However, with AI-driven analytics, product groups can now advantage real-time insights from this facts deluge.
These insights can uncover patterns that human analysts might forget, guiding product groups to make more knowledgeable choices. Whether it is identifying which features customers interact with maximum or pinpointing regions of improvement, AI equips product teams with a granular know-how of their product's overall performance in actual-global situations.
3. Enhanced User Experience Through Personalization
In the era of consumer-centricity, personalization has become paramount. AI performs a pivotal role in this. By reading person conduct, preferences, and feedback, AI fashions can tailor product experiences for individual users or companies.
For instance, an AI-driven CRM might automatically segment contacts based on interaction history or suggest communication strategies tailored to specific client personas. This level of personalization, powered by way of AI, results in merchandise that resonate extra deeply with customers, fostering loyalty and engagement.
4. Predictive Analysis for Proactive Product Evolution
One of the standout capabilities of AI is its predictive prowess. Instead of simply reacting to trends or issues, product teams can leverage AI's predictive analytics to anticipate future needs or challenges.
Imagine a B2B SaaS platform that, through AI, identifies a potential scalability issue due to a predicted surge in user numbers six months down the line. With this foresight, developers can proactively decorate the platform's infrastructure, ensuring seamless overall performance whilst person numbers do in the end spike.
5. Continuous Learning and Adaptation
Traditional software, once developed, remains largely static until the next update or version. AI-driven products, on the other hand, are dynamic by nature. Machine studying fashions, a subset of AI, research and adapt based totally on new information and interactions. This means that the product evolves and improves continuously, without watching for manual updates.
For companies, this represents an ever-evolving tool that grows and adapts with their needs, ensuring sturdiness and sustained relevance in a fast-paced virtual landscape.
3. The Intersection of AI and Information Security
In the technology of burgeoning virtual transformation, the mixture of Artificial Intelligence (AI) with Information Security has emerged as both an exciting prospect and a frightening project. The alliance of those domains holds great promise, but it also introduces complexities that tech specialists need to navigate astutely. Delving deep into this intersection, permit's explore the transformative capacity and the associated risks.
1. AI as the Vanguard of Security Operations
- Real-time Threat Detection: Traditional security systems often relied on predefined rules and heuristics. AI, with its gadget mastering talents, can examine giant quantities of records at lightning pace, detecting anomalies and capacity threats in real-time. This proactive method guarantees that breaches can be recognized and mitigated earlier than they improve.
- Predictive Analytics: Beyond just real-time detection, AI can predict potential vulnerabilities by analyzing patterns and trends. By expertise the modus operandi of cybercriminals, AI-pushed structures can make stronger defenses in anticipation of future attacks.
- Phishing Detection: AI algorithms can swiftly scan emails and websites, identifying subtle signs of phishing attempts that might elude human eyes, thus protecting users from potential scams.
2. Challenges and Vulnerabilities Introduced by AI
- Adversarial Attacks: As AI models become integral to security, they become targets themselves. Adversarial assaults contain feeding deceptive statistics to AI systems to trick them, doubtlessly inflicting them to misclassify or overlook threats.
- Data Dependency: AI's strength is its ability to analyze data. However, this is a double-edged sword. If the data used to train AI models is biased or flawed, it can lead to skewed security decisions, creating potential vulnerabilities.
- Complexity: AI models, especially deep learning networks, are complex. This complexity can on occasion be a barrier, making it hard for security experts to recognize or interpret certain selections made by way of the system.
3. Ethical and Regulatory Implications
- Surveillance Concerns: AI's capability to monitor and analyze can easily slip into the realm of excessive surveillance, raising privacy concerns. Businesses want to strike a stability, making sure protection with out infringing on man or woman privateness.
- Regulatory Compliance: As governments and establishments grapple with the results of AI, policies are evolving. For corporations, this indicates they ought to make certain that their AI-pushed security measures follow local and worldwide legal guidelines, which could now and again be a transferring goal.
4. Embracing a Collaborative Approach
It's essential to understand that while AI offers powerful tools in the realm of information security, human oversight remains crucial.
- Human-in-the-loop: This approach ensures that while AI can detect and even predict threats, critical decisions, especially those with significant ramifications, have a human touch. It's a synergy where AI provides the computational prowess, and humans bring judgment and contextual understanding.
- Continuous Training: The cyber landscape is ever-evolving, with new threats emerging daily. AI models need continuous schooling with the ultra-modern records to live ahead of cyber adversaries.
4. How Tech Industry Professionals are Adapting to the AI Trend
The infusion of Artificial Intelligence (AI) into the tech landscape is akin to the introduction of electricity in the previous centuries - it's not just a change, it's a revolution. As AI continues its speedy ascent, specialists inside the tech realm, especially those connected with SaaS and B2B software program, are not just spectators; they are energetic individuals on this transformative journey. Here's a closer look at how they are adapting:
1. Lifelong Learning and Upskilling
The modern tech professional recognizes that learning is an ongoing process. Traditional coding skills, while still vital, are being supplemented with expertise in machine learning, neural networks, and data analytics.
- Courses and Certifications: Platforms like Coursera, Udemy, and edX offer specialized courses on AI and machine learning. These guides, regularly taught through enterprise professionals, provide both theoretical knowledge and sensible capabilities.
- Workshops and Bootcamps: Many professionals are attending intensive bootcamps, which provide hands-on experience in building and deploying AI models.
2. Cross-disciplinary Collaboration
The siloed approach of yesteryears is giving way to a more integrated, collaborative work environment.
- Data Scientists and Developers: Instead of running in isolation, information scientists are actually operating shoulder-to-shoulder with builders, making sure that AI fashions are seamlessly incorporated into software program solutions.
- Business Analysts and AI Experts: Business requirements are being melded with AI capabilities, with analysts working closely with AI experts to craft solutions that are both technically sound and business-relevant.
3. Engaging in Ethical Debates
AI, with its vast potential, brings forth ethical dilemmas. Tech professionals are now not just passive coders; they're actively engaged in discussions and debates about the ethical implications in their work.
- Bias and Fairness: There's a growing awareness about unintentional biases in AI models, leading to a push for more transparent and fair algorithms.
- Privacy Concerns: Data is the lifeblood of AI, but professionals are deeply concerned about ensuring it's used responsibly, maintaining user confidentiality and trust.
4. Networking and Community Building
Given the rapid evolution of AI, staying updated is a challenge. Professionals are countering this by building strong networks and communities.
- AI Conferences and Summits: Events like NeurIPS, ICML, and GTC are seeing a surge in attendees, not just from academia but also from the industry. These events are platforms for knowledge exchange, discussions, and collaborations.
- Online Forums and Groups: Platforms like Stack Overflow, Reddit's Machine Learning community, and others are buzzing with discussions, queries, and shared resources. They act as real-time knowledge banks and support systems.
5. Embracing New Tools and Platforms
The AI atmosphere is wealthy with tools and systems designed to simplify development and deployment.
- Cloud Platforms: Tech professionals are rapidly adopting cloud platforms like AWS SageMaker, Google AI Platform, and Azure Machine Learning, which offer tools to build, train, and deploy AI models at scale.
- Open-source Libraries: Libraries like TensorFlow, PyTorch, and Keras are becoming staples in a developer's toolkit, offering pre-built functions and structures for efficient AI model development.
5. Challenges and Considerations in AI-Driven SaaS Development
The transformative power of AI in B2B SaaS development is undeniable. However, the integration of artificial intelligence into software-as-a-service platforms isn't a mere plug-and-play affair. Deep complexities arise, each demanding thoughtful consideration to ensure that the promise of AI doesn't turn into a Pandora's Box. Below are some of the paramount challenges and considerations developers, businesses, and stakeholders must grapple with:
1. Data Privacy and Security Concerns
The Heart of AI: AI's efficacy is largely rooted in its ability to analyze massive volumes of data. This raises immediate questions about data acquisition, storage, and usage.
Regulatory Hurdles: With regulations like the General Data Protection Regulation (GDPR) and the California Consumer Privacy Act (CCPA), businesses have to tread carefully. Compliance is not pretty much averting fines; it is about preserving patron trust.
Breaches: An AI system with access to vast data, if compromised, can lead to monumental breaches. Safeguarding these systems becomes paramount.
2. Bias and Ethical Dilemmas
Unintended Prejudices: AI models can magnify human biases present in their training data. For instance, a recruitment tool trained on data from an industry with gender disparities might inadvertently favor one gender over another.
Transparency and Accountability: If an AI system makes a flawed decision, who's to blame? The developers? The trainers? The data providers? This murky territory needs clear delineation.
3. High Initial Investment
Talent Acquisition: Finding the right talent versed in AI can be costly. Not only are salaries for AI specialists high, but the competition for seasoned experts is fierce.
Infrastructure Costs: Running AI models, especially during the training phase, can demand significant computational resources, leading to increased costs.
4. Integration Complexities
Legacy Systems: For businesses with pre-existing software infrastructures, integrating new AI tools can be akin to changing the engine of a running car. The transition can be slow, complex, and riddled with compatibility issues.
User Adaptation: Even the most advanced AI tool is ineffective if end-users can't, or won't, use it. Ensuring intuitive user experiences while introducing new AI functionalities is a balancing act.
5. Continuous Evolution and Maintenance
Shifting Goalposts: Unlike traditional software, which might see periodic updates, AI models can require frequent retraining, especially when the underlying data or business goals change.
Dependence on Quality Data: AI's performance is directly tied to the quality of data it's fed. Inconsistent or inaccurate data can drastically diminish the model's reliability and outputs.
6. Ethical Use of AI
Moral Implications: Beyond biases, there's a broader moral question about how AI should be used. Should an AI tool, for instance, be used to monitor employee productivity or personal behaviors? Where's the line between helpful and intrusive?
6. Strategies for Successful AI Implementation in B2B SaaS
The integration of Artificial Intelligence (AI) into B2B SaaS solutions is undeniably transformative. However, its a success deployment calls for a strategic technique that is each comprehensive and nuanced. Here, we delve deeper into effective techniques that corporations ought to consider:
1. Understand Your Business Objectives
Before diving into AI, it is vital to have a clean know-how of what you want to acquire. Is it customer retention? Predictive analytics? Streamlining operations?
- Set Clear Goals: Establish well-defined, measurable objectives. For instance, "We aim to improve customer response time by 20% using an AI-driven chatbot."
- Align with Business Strategy: Ensure that your AI initiatives align with your broader business goals and vision. The technology have to be a device to propel your commercial enterprise forward, no longer just a buzzword.
2. Start Small and Scale Gradually
Instead of aiming for massive, resource-intensive projects, initiate with modest, manageable AI projects.
- Pilot Projects: Test AI implementations on smaller datasets or a subset of your users. This lets in you to gauge its efficacy and make adjustments earlier than a complete-scale rollout.
- Iterative Approach: Learn from each project, making necessary adjustments, and then move on to larger, more complex projects.
3. Collaborate with AI Experts
While it's tempting to jump on the DIY bandwagon, AI is complex. Partnering with experts can significantly boost your success rate.
- External Consultants: Seek guidance from AI consultancies or specialists who have a track record in your sector.
- In-house Talent: Consider hiring or training in-house AI specialists. They can provide insights tailor-made in your particular commercial enterprise desires and industry nuances.
4. Focus on Data Quality
AI is only as good as the data it's fed. Ensuring data quality is paramount.
- Data Cleaning: Regularly audit and clean your data to remove inaccuracies and inconsistencies.
- Data Protection: With data breaches becoming increasingly common, prioritize security measures to protect your data. This now not handiest ensures the integrity of your AI models however also fosters agree with together with your stakeholders.
5. Ethical Considerations and Transparency
In the age of data, trust is paramount. Users and stakeholders want to understand how AI-driven decisions are made.
- Transparent Algorithms: While proprietary tech is treasured, provide insights into how your AI makes decisions, particularly in vital regions like finance or healthcare.
- Ethical Guidelines: Develop a set of ethical guidelines for your AI implementations. This should address concerns like data privacy, bias mitigation, and more.
6. Continuous Training and Updates
The world of AI is dynamic. Models that were efficient a year ago might be obsolete today.
- Ongoing Training: Regularly feed your AI models with fresh data to ensure they remain relevant and efficient.
- Stay Updated: The AI network is vibrant, with new studies and techniques rising continuously. Stay updated with the contemporary advancements to make certain your implementations continue to be current.
7. Foster a Culture of AI Acceptance
Change, especially one as transformative as AI, can be daunting for employees.
- Training Sessions: Organize workshops and training sessions to familiarize your team with AI tools and processes.
- Open Communication: Encourage feedback and discussions approximately AI. Address concerns, dispel myths, and spotlight the benefits of AI-driven procedures.
7. Expert Opinions on AI in B2B SaaS
Several tech luminaries have weighed in on the topic. Let's hear from them:
"In the age of digital transformation, AI stands out not just as a disruptor but as an enabler. The B2B SaaS sector is just scratching the surface of what's possible," remarks Jane Smith, a renowned SaaS entrepreneur.
"Security in an AI-first world demands a proactive rather than a reactive approach. The stakes are high, but so are the rewards," opines Robert Lee, a leading voice in information security.
8. A Look Ahead: Predictions for AI and B2B SaaS
The confluence of AI and B2B SaaS represents a pivotal second for the tech enterprise. As these technology become similarly entwined, their mutual evolution guarantees to reshape now not just how corporations function, however additionally how they conceive and set up answers. Let's delve deeper into the transformative capacity looking forward to us.
1. Emergence of AI-Powered Collaboration Tools
Collaboration has always been the linchpin of successful businesses. As far flung work turns into a mainstay, there is an increasing demand for equipment that not simply facilitate, however increase collaboration.
- Smart Meeting Schedulers: Moving beyond mere calendar coordination, AI-driven tools can understand participants' work habits, time zones, and preferences to schedule meetings that suit everyone. These tools might even factor in predicted mental fatigue based on a participant's prior commitments or daily routine.
- Real-time Content Collaboration: Imagine working on a document or a design and having AI suggest changes, improvements, or alternatives in real-time, drawing upon a vast database of industry best practices.
- Emotionally Aware Communication Platforms: Future video conferencing tools might employ AI to gauge the emotional tone of a meeting, offering real-time feedback to participants or even suggesting breaks if a discussion becomes too heated.
2. Personalized B2B Experiences
The era of one-size-fits-all solutions is waning. Personalization, often seen in B2C platforms, will become a hallmark of B2B SaaS, fundamentally changing the client-provider relationship.
- Adaptive Software Interfaces: B2B software might soon adapt to the individual user. For example, an analytics tool could prioritize certain data visualizations based on a user's role or past interactions.
- Predictive Problem-Solving: AI can analyze patterns to predict potential issues a business might face. B2B solutions could then proactively offer tools, resources, or support even before a problem manifests.
- Custom Learning Pathways: As SaaS platforms become more intricate, onboard training will be tailored. AI will create mastering pathways primarily based on an employee's present know-how, position, and learning pace.
3. Ethical AI Frameworks in B2B SaaS
As AI's footprint expands, so does the moral and ethical responsibility of companies employing it. The B2B SaaS industry will likely be at the forefront of defining and adopting ethical AI standards.
- Transparent Algorithms: Businesses will demand clarity on how AI-driven tools make decisions, leading to the development of transparent or "explainable" AI models.
- Bias Audits: With the recognition that AI can perpetuate biases, third-party audits of AI algorithms will become standard. This will ensure that AI tools in B2B SaaS are equitable and fair.
- Ethical Consulting: Just as businesses today hire consultants for cybersecurity, the future will see a rise in AI ethics consultants. These professionals will guide businesses in developing and deploying AI responsibly.
9. Case Studies: SaaS Companies Leveraging AI
The practical applications of AI in the B2B SaaS realm offer a tangible glimpse into the transformative power of this technology.
1. OptiSoft: Enhancing CRM through AI
Background: OptiSoft, a leading CRM solution provider for small and medium businesses, faced a challenge. While their platform boasted a range of features, users found it difficult to navigate and leverage these tools efficiently, leading to underutilization.
AI Implementation: The company decided to integrate an AI-driven virtual assistant into their platform. This assistant was designed to:
- Understand user queries in natural language
- Offer real-time guidance on using the platform's features
- Analyze user behavior to predict and proactively offer support
Results: Within six months of integrating the AI assistant:
- User engagement with the platform's features increased by 45%.
- Customer support tickets related to navigation decreased by 70%.
- Client feedback indicated a heightened sense of satisfaction with the platform's user-friendliness and intuitive design.
2. AnalyzrPro: AI-Driven Real-Time Business Analytics
Background: AnalyzrPro, a budding SaaS startup, aimed to provide businesses with real-time analytics that could inform strategic decisions. However, with the vast amounts of data businesses deal with, providing actionable insights in real time was a challenging feat.
AI Implementation: To address this, AnalyzrPro employed deep learning models to:
- Continuously analyze incoming data streams.
- Detect patterns, anomalies, and trends.
- Present these insights through a visually intuitive dashboard.
Additionally, their system was designed to learn from user interactions. Over time, it began to highlight insights that were most relevant to specific business roles, from marketing managers to CFOs.
Results: With their AI-driven approach:
- Clients reported a 35% reduction in the time taken to derive actionable insights from data.
- The platform saw a 25% increase in user logins, indicating higher engagement.
- 80% of surveyed clients stated that the AI-driven insights influenced their strategic decisions.
3. SafeGuard: AI-Powered Cybersecurity for SaaS
Background: In an era where data breaches are increasingly common, SafeGuard, a cybersecurity SaaS solution, aimed to offer businesses an edge in data protection.
AI Implementation: SafeGuard incorporated AI to:
- Monitor network traffic in real-time, detecting anomalies indicative of potential threats.
- Employ machine learning to discern between false alarms and genuine threats, reducing alert fatigue.
- Automatically update threat detection algorithms based on emerging global cybersecurity threats.
Results: Post AI integration:
- SafeGuard's clients experienced a 60% reduction in security breaches.
- IT teams reported a 40% reduction in time spent analyzing and responding to potential threats.
- The platform received endorsements from several industry leaders, cementing its reputation as a pioneer in AI-driven cybersecurity.
10. FAQ
Q1: Why is AI integration becoming crucial for B2B SaaS companies?
A1: AI integration allows B2B SaaS companies to offer enhanced user experiences, automate repetitive tasks, and derive insights from vast amounts of data. This now not simplest improves performance but additionally allows in turning in personalized answers, leading to better purchaser pride and retention.
Q2: What challenges might a SaaS company face when trying to integrate AI into their platform?
A2: Companies might face challenges such as high initial costs, data privacy concerns, potential biases in AI models, and complexities in integrating AI tools into existing systems. Ensuring that the AI is moral and abides by way of regulatory requirements is likewise vital.
Q3: How can SaaS companies ensure the ethical use of AI?
A3: Companies can prioritize transparency, allowing users to understand how AI decisions are made. Regularly auditing and updating AI models to avoid biases, and adhering to standardized ethical guidelines can also guide the ethical use of AI.
Q4: Are there specific sectors within B2B SaaS that benefit more from AI integration?
A4: While AI offers advantages across the B2B SaaS spectrum, sectors that deal with vast amounts of data like CRM, business analytics, and cybersecurity often see immediate and significant benefits from AI integration.
Q5: How does AI affect the job landscape within SaaS companies?
A5: AI can automate certain tasks, but it also creates new roles and opportunities. There's a developing demand for AI specialists, information scientists, and experts who can bridge the distance among conventional software development and AI integration.
Q6: Can smaller SaaS startups afford to integrate AI into their platforms?
A6: Yes, while AI implementation can be resource-intensive, there are cost-effective solutions, tools, and frameworks available. Starting small, perhaps with a pilot project, and scaling up can make AI integration feasible for startups.
Q7: How do B2B SaaS companies ensure data security when using AI models?
A7: Companies can prioritize end-to-end encryption, employ AI-powered cybersecurity tools for real-time threat detection, and regularly update their AI models and systems to combat emerging security threats.
Q8: With the increasing use of AI, how can companies differentiate themselves in the market?
A8: Differentiation can come from the unique application of AI, focusing on niche problems, ensuring the ethical use of AI, offering top-tier user experiences, and providing exceptional post-sale support and continuous learning opportunities.
11. Conclusion
In the vast and ever-evolving panorama of B2B SaaS, the integration and utilization of AI stand out as a beacon of transformative ability. As our exploration shows, from optimizing consumer reviews to deriving actionable commercial enterprise insights and fortifying cybersecurity, AI isn't always only a supplementary tool however a sport-changer.
Yet, as with any effective technology, it comes with its challenges. Navigating the geographical regions of statistics privacy, ensuring the moral software of AI, and tackling integration complexities are only a few hurdles agencies may face. However, the rewards – in terms of efficiency, user satisfaction, and innovation – far outweigh the potential pitfalls.
The case research, FAQs, and insights shared in this discourse remove darkness from a novel fact: AI is not merely a trend however a paradigm shift. As B2B SaaS companies, from fledgling startups to established giants, grapple with this shift, one thing is certain. Those that embrace AI, wield it responsibly, and continuously innovate will not only survive but thrive in this brave new world.
For industry professionals, be it in information security, SaaS development, or any other tech niche, the message is unambiguous. The future is AI-augmented, and it beckons with promise and potential. As we stand on this precipice of technological evolution, it's now not pretty much keeping tempo but envisioning and shaping the destiny we need.
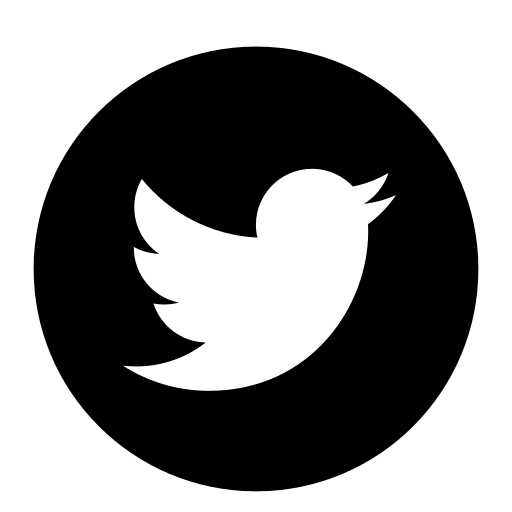
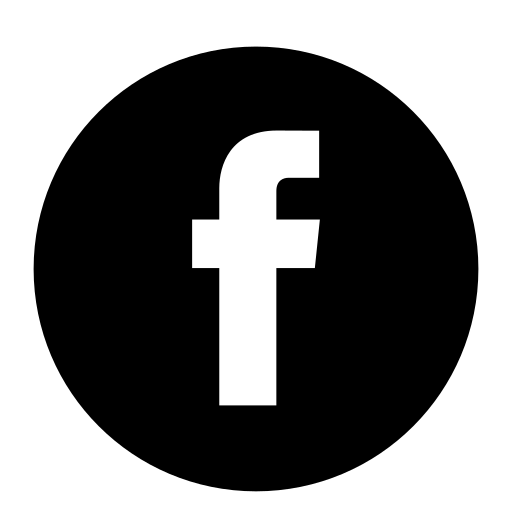
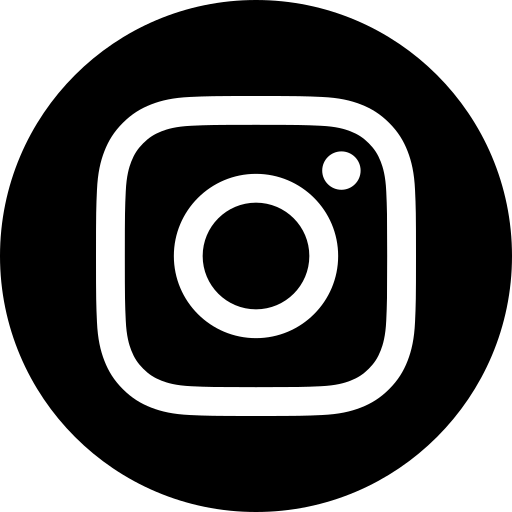