Ethical AI: Navigating the Moral Landscape of AI in Financial Services

1. Introduction: The Rise of AI in Financial Services
In current years, the monetary offerings enterprise has witnessed a large surge within the application of artificial intelligence (AI). From predictive analytics in funding strategies to chatbots for customer support, the potential of AI appears countless. But with extraordinary power comes incredible duty. As those technology emerge as deeply ingrained in our financial structures, the question of ethics involves the leading edge.
2. Ethical Dilemmas in AI: The New Challenge
In the burgeoning international of synthetic intelligence, the charm of modern-day technological advancements often captures our collective creativeness. But, as with any transformative power, AI brings with it a fresh set of ethical conundrums that cannot, and should not, be overlooked. In the context of monetary services, those moral dilemmas are in particular salient, given the massive influence this zone has on economies, groups, and individual lives.
Bias in AI Algorithms
AI systems learn from vast quantities of data, often ingesting historical information to predict future patterns. While this is a strength of machine learning, it's also its Achilles heel. If the data is tainted with historical prejudices, biases, or inaccuracies, the AI will perpetuate these biases in its outputs. For instance, if a bank's past loan approval data reflects a prejudice against a certain demographic, an AI trained on this data might decline loans to this group at a disproportionately high rate.
Bias in AI is not just an issue of fairness; it can also lead to poor business decisions. By not comparing mortgage candidates based totally on goal financial criteria, the bank ought to pass over out on worthwhile lending opportunities.
Transparency: The 'Black Box' Dilemma
One of the most common criticisms of AI, particularly deep learning models, is their 'black box' nature. Even when they are effective, it's often not clear how they arrived at a particular decision. In financial services, this is problematic. If an investment strategy driven by AI goes awry or a customer is denied a loan, stakeholders will demand explanations. And "the AI decided so" is hardly a satisfactory answer.
Regulations like the European Union's General Data Protection Regulation (GDPR) have already recognized this challenge, enshrining the 'right to explanation' for AI decisions in law. Financial institutions must thus prioritize AI models that offer interpretability and can provide a clear rationale for their decisions.
Privacy Concerns in an Age of Big Data
The insatiable urge for food of AI for facts has intensified concerns around privateness. As financial institutions collect and analyze more detailed data about customers – from spending habits to social media activity – the potential for abuse or inadvertent data breaches magnifies. Ethical AI must respect user privacy, using data judiciously and safeguarding it rigorously. This is not just a moral imperative but also a business one. In a world where data breaches make headlines, and regulatory fines can run into billions, privacy cannot be an afterthought.
Reliability and Accountability
As AI structures end up extra autonomous, questions around reliability and accountability grow to be paramount. If an AI-driven trading system makes a poor decision that results in significant financial losses, who is responsible? The software developers? The data scientists who trained the model? The executives who approved its use? The challenge is twofold: ensuring AI systems are reliable and, when things go wrong, having clear lines of accountability.
3. The Role of Information Security in Ethical AI
In today's digitized financial landscape, the integration of artificial intelligence (AI) is no longer a distant vision but a present reality. AI's unprecedented computational power can sift through big facts sets, expect market tendencies, automate trading, customize banking reviews, and plenty extra. Yet, as with all powerful tools, the deployment of AI brings with it an assortment of ethical considerations. One of the most paramount among these is the role of information security.
The Convergence of AI Ethics and Information Security
At first glance, the domains of AI ethics and information security may seem distinct. However, a closer examination reveals their deeply intertwined nature, particularly in the monetary sector. Here's why:
- Data Integrity: AI systems are only as good as the data they're fed. If this data is compromised, biased, or tampered with, the results can be not only inaccurate but also unethical. Information safety guarantees that statistics stays untouched and uncorrupted, making sure AI fashions make selections based on factual, unbiased facts.
- Confidentiality: The financial sector handles some of the most sensitive data, from personal identification details to transaction histories. AI models that process this data must do so with utmost discretion. Robust information safety features make sure that this information stays personal, preventing breaches that would result in moral and legal repercussions.
- Transparency and Accountability: An essential facet of ethical AI is the ability to trace back and understand how a particular AI decision was made. Information security equipment, such as secure logging and tamper-evidence audit trails, play a pivotal role in supplying this transparency. They ensure that every step inside the AI selection-making manner is recorded securely, fostering agree with and accountability.
The Challenges Ahead: New Frontiers in AI and Information Security
With the increasing complexity and class of AI fashions, particularly deep gaining knowledge of algorithms, the demanding situations for data safety develop exponentially.
- Adversarial Attacks: These are sophisticated techniques where malicious actors introduce subtle, almost indistinguishable alterations to input data, causing AI models to make incorrect decisions. For financial institutions, this will imply faulty investments or erroneous approvals of high-threat loans. Information protection has to evolve to hit upon and shield against such nuanced threats.
- Data Poisoning: This is a tactic where attackers introduce false data into the system, corrupting the data pool that trains the AI. The repercussions may be long-lasting, as the AI usually learns from this tainted data. Effective statistics safety features should be in region to validate and confirm facts assets, making sure they may be true and untampered.
Safeguarding the Ethical Promise of AI
The promise of AI in financial services is profound. It heralds a destiny of customized banking, optimized investments, and enhanced patron reviews. Yet, this promise can only be realized—and remain ethical—if underpinned by robust information security.
In essence, for economic institutions to wield AI responsibly, they ought to view statistics protection no longer just as a technical requirement however as an ethical imperative. As we circulate deeper into the AI-driven era, this synergy between AI ethics and information protection may be the linchpin making sure that era serves humanity with integrity, recognize, and fairness.
4. How B2B SaaS is Shaping Ethical Standards in AI
In today's rapidly digitalizing world, the significance of B2B Software as a Service (SaaS) platforms cannot be understated. These structures are at the forefront of innovation, specially within the sphere of synthetic intelligence. But past simply facilitating AI's capabilities, they play a pivotal role in shaping its ethical obstacles. Let's dive deeper into the impact of B2B SaaS on the moral requirements of AI, particularly within the monetary domain.
A Framework for Trust
B2B SaaS companies operate on a foundational principle: trust. Their business model necessitates maintaining the confidence of other businesses, often over long-term relationships. This trust factor is amplified when AI is in play, given the uncertainties and concerns surrounding its use.
To ensure this trust, many SaaS providers have started to incorporate:
- Ethical Guidelines and Toolkits: These are resources that guide users on how to utilize AI tools ethically, ensuring that biases, privacy invasions, and other unethical practices are minimized or eradicated.
- Transparent Algorithms: They offer solutions that let businesses 'peek' behind the AI curtain, understanding how decisions are being made. This transparency is critical for ethical validation and stakeholder trust.
The Democratization of Ethical Practices
One of the hallmarks of SaaS platforms is accessibility. By providing AI tools and offerings on a scalable, subscription foundation, they may be democratizing get entry to to fine practices in AI ethics. Smaller financial institutions, which might not have the resources to develop ethical AI systems from scratch, can leverage these platforms to ensure their practices are up-to-date and in line with industry standards.
Continuous Learning and Adaptation
The nature of SaaS is iterative. Constant updates, improvements, and feedback loops are intrinsic to this model. This continuous evolution is beneficial for ethical AI standards, as:
- Feedback is Swift: If an ethical issue arises, it can be flagged, addressed, and rectified in real-time.
- Collaborative Ethical Development: With multiple clients from diverse sectors using the platform, B2B SaaS providers can draw from a wide range of ethical challenges and solutions, refining their tools accordingly.
Setting the Gold Standard
Several leading B2B SaaS providers have taken it upon themselves to not just adhere to ethical AI standards, but to define them. By participating with enterprise corporations, moral researchers, and AI professionals, they are developing the tips with the intention to shape the destiny of AI inside the financial quarter and past.
Collaborative Ecosystems for Ethical Progress
It's not just about the SaaS providers and their direct clients. An ecosystem is forming, consisting of third-party developers, integrators, and other stakeholders, all collaborating on the SaaS platform. This environment promotes shared moral values, ensuring that as AI programs make bigger, they do so within a framework that prioritizes ethical issues.
5. AI Ethics Committees: Paving the Way
In an era where artificial intelligence (AI) permeates numerous facets of the financial sector, the emergence of AI ethics committees within organizations has become more than just a trend—it's a necessity. Let's take a more in-depth look into why these committees are so pivotal, what they bring about to the desk, and the profound effect they can have on shaping the ethical panorama of AI in economic services.
Why the Need for AI Ethics Committees?
Navigating the Gray Areas: AI, while revolutionary, often operates in ethical gray zones. Human biases can creep into AI models, decisions made by AI can sometimes lack transparency, and data privacy is a constant concern. Here is where ethics committees step in, offering guidance, setting boundaries, and ensuring adherence to ethical norms.
Stakeholder Trust: Trust is the bedrock of the financial industry. Clients, partners, and stakeholders want guarantee that AI-driven financial choices are independent, truthful, and obvious. An ethics committee serves as a testimony to an enterprise's dedication to these concepts.
Composition of an AI Ethics Committee
A successful AI ethics committee is often multidisciplinary, comprising:
- Technical Experts: Those who understand the intricacies of AI algorithms, data science, and machine learning.
- Ethicists: Individuals trained in ethics, philosophy, or related disciplines, who can guide discussions on moral implications and the broader societal impact of AI choices.
- Industry Veterans: Professionals with deep roots inside the economic region, supplying context on how AI packages align with industry requirements and practices.
- External Consultants: Often, fresh perspectives from external AI or ethics experts can provide unbiased insights and recommendations.
Key Responsibilities and Actions
Regular Audits: One of the primary tasks of an AI ethics committee is to conduct regular audits of AI models and applications. This ensures that algorithms continue to be unbiased, choices are transparent, and information use aligns with ethical and legal norms.
Guideline Formulation: The committee is also responsible for drafting, updating, and enforcing ethical guidelines specific to AI applications within the organization. These recommendations act as a roadmap for developers, statistics scientists, and different stakeholders.
Staying Updated: The world of AI is ever-evolving. Ethics committees must continually replace themselves with the trendy in AI research, ethical debates, and enterprise exceptional practices. This regularly entails attending meetings, workshops, or participating with instructional establishments.
Conflict Resolution: If disagreements or conflicts arise within the organization regarding AI applications, the ethics committee acts as an arbitrator, ensuring that decisions align with the organization's ethical stance.
Stakeholder Engagement: Effective communication with internal teams, clients, and the broader public is crucial. The committee regularly plays a role in explaining AI selections, assuaging concerns, and gathering comments to refine ethical recommendations.
Challenges Faced by AI Ethics Committees
While the path is noble, it isn't without challenges. Some of the hurdles faced by ethics committees include:
- Striking a balance between technological innovation and ethical considerations.
- Addressing the 'black box' nature of certain AI models, which can be challenging to interpret.
- Navigating global differences in ethical norms and regulatory guidelines.
6. Collaborative Approaches: The Way Forward
In the vast and ever-evolving expanse of the financial sector, the integration of Artificial Intelligence (AI) poses a labyrinth of ethical dilemmas and technical quandaries. In facing these challenges, siloed efforts are not merely insufficient—they’re fundamentally misaligned with the interconnected, interdependent nature of modern financial ecosystems.
The Essence of Collaboration in Ethical AI Adoption
In embracing AI, financial institutions are not merely adopting a technology; they are inviting an entity that will inevitably impact decision-making processes, customer interactions, and overall business strategies. The endeavor to harness AI ethically, then, is not only a technical assignment but a holistic one, spanning throughout numerous elements of operations and impacting several stakeholders.
Collaboration, specially with B2B SaaS providers, presents a unison wherein information meets innovation, a crossroads where ethical concepts intertwine with superior technology.
- Expertise: B2B SaaS providers specializing in ethical AI solutions offer a reservoir of knowledge and experience that is crucial for navigating through the ethical complexities and technical nuances of AI integration.
- Technological Innovation: These providers are not just vendors; they are innovators who have their fingers perpetually on the pulse of technological advancements, ensuring that the solutions offered are not just compliant with current norms but are also forward-compatible with emerging trends and regulations.
- Scalable Solutions: SaaS platforms often provide scalable solutions that can adapt and grow with the financial institution, ensuring that the ethical AI frameworks and mechanisms in place can handle future expansions and diversifications seamlessly.
Building a Networked Ecosystem of Ethical AI Practice
A symbiotic relationship between financial entities and B2B SaaS providers creates a networked ecosystem where ethical AI practices can be cultivated, nurtured, and proliferated. The collaborative technique goes beyond the limits of man or woman organizations, fostering a community in which insights, demanding situations, answers, and improvements are shared and jointly enriched.
- Shared Knowledge: Collaboration fosters an surroundings in which understanding isn't hoarded however shared, ensuring that improvements and insights gain the broader economic network, uplifting the enterprise as a whole.
- Collective Mitigation of Risks: In an interconnected financial environment, the ripple effects of ethical missteps in AI can traverse through the entire industry. Collaborative methods facilitate collective threat mitigation, safeguarding now not just person entities but the financial region as an entire.
- Unified Standards: Through collaboration, institutions, and providers can work towards establishing unified standards of ethical AI, ensuring that the financial sector progresses cohesively towards responsible AI adoption.
The Future Shaped by Collective Wisdom and Shared Ethical Values
In the collective pursuit of ethically harnessing AI, the financial sector does not merely safeguard its own interests, but it also contributes towards shaping a future where technology and ethics coexist harmoniously. The collaboration between economic establishments and B2B SaaS companies as a consequence will become a beacon that illuminates the route ahead, guided by shared ethical values and empowered via collective expertise and collaborative improvements.
"In the melding of technology and ethics, let us find not just solutions, but also discover our shared values and collective aspirations." - Alexandra T. Freeman, Author of 'Navigating AI Ethics in Finance'.
The journey towards ethical AI is indeed challenging but remember that in collaboration, there is not just strength, but also wisdom, innovation, and a shared commitment towards a future that honors ethical values and technological advancements alike.
7. Practical Steps to Implementing Ethical AI in Financial Services
As we delve deeper into the world of AI ethics, it is crucial for decision-makers to have actionable steps they can observe to make certain they're at the proper tune. Here's a concise guide:
- Identify Ethical Goals: Before diving into AI applications, pinpoint what ethical AI means for your institution. This could be transparency, fairness, privacy, or a combination of these and more.
- Diverse Data Sets: Ensure the data sets used to train AI models are diverse, representing all customer demographics to prevent biases.
- Continual Monitoring: AI is not a set-it-and-forget-it tool. Continuously monitor AI applications to ensure they maintain ethical standards over time.
- Stakeholder Engagement: Regularly communicate with stakeholders, including customers, about how AI is being used and the measures in place to ensure its ethical use.
- Collaborate with Experts: As mentioned, partnering with B2B SaaS providers can offer invaluable insights and tools for your ethical AI journey.
- Transparency in Algorithms: Where possible, use transparent algorithms or provide explanations for AI-driven decisions.
- Ethical AI Training: Ensure that all team members, especially those directly interacting with AI tools, receive training on the ethical implications and use of AI.
8. Feedback Loop: Learning from Mistakes
In the dynamic world of AI within financial services, the journey toward ethical implementations is as much about introspection as it is about innovation. The comments loop, particularly when mastering from missteps, is a pivotal mechanism that ensures AI systems evolve whilst staying rooted in moral issues. Delving deeper into this, we find the complicated layers that make a remarks loop not simply useful, but crucial.
Why Feedback Loops Matter
Mistakes are a natural part of progress. In an area as difficult and novel as AI, those missteps are not simply expected—they are invaluable mastering opportunities. However, the important thing lies in efficiently harnessing those instructions. A feedback loop ensures that insights won from those errors are systematically fed again into the AI device, refining and optimizing it for future operations.
Key Components of an Effective Feedback Loop
- Recognition: Before rectification, there must be recognition. Financial establishments should appoint sturdy monitoring gear to discover anomalies, biases, or any deviations from anticipated AI behavior.
- Open Channels: Create an environment where both employees and customers can voice concerns or observations. This may involve anonymous reporting tools or dedicated feedback platforms for AI-driven services.
- Analysis: Every piece of feedback, whether from internal monitoring or external channels, should be thoroughly analyzed. Dive deep into the root causes. Was it a data issue, an algorithmic bias, or perhaps a lack of clarity in AI decision-making?
- Implementation: Post-analysis, the insights must translate into actionable changes. This could be in the form of tweaking algorithms, sourcing more diverse data sets, or even retraining the AI model from scratch.
- Communication: After implementing changes, communicate them. Let stakeholders recognize that their remarks turned into valuable and elucidate on the measures taken to rectify troubles.
Real-world Application: A Case in Point
Consider a scenario where a bank's AI-driven loan approval system inadvertently displays bias against applications from a particular demographic. Once this issue surfaces—either through internal checks or external feedback—the bank's first step would be to acknowledge and halt the problematic operation.
The feedback loop then kicks in:
- The bank analyzes vast amounts of decision data to pinpoint the source of the bias.
- Simultaneously, they open channels for affected customers to voice their concerns or share their experiences.
- With a combination of customer feedback and data analysis, the bank identifies the flawed algorithmic component.
- The system is retrained, tested, and validated to ensure unbiased decisions.
- Finally, the bank communicates its corrective actions, assuring stakeholders of its commitment to ethical AI.
Embracing the Loop
The feedback loop isn't a one-time process. It's continuous and cyclical. As AI technology and packages grow, so will the challenges. The loop guarantees that financial institutions stay agile and adaptive, constantly ready to learn, iterate, and improve.
9. Looking Ahead: The Future of Ethical AI in Finance
As AI technologies continue to adapt, so will the ethical challenges related to them. Financial institutions that prioritize ethics now will be better positioned to navigate future challenges. With the proper stability of technology, human oversight, and a stable moral framework, the destiny of finance looks both promising and principled.
"In the world of finance, where trust is currency, ethical AI is not just an option—it's an imperative." - Dr. Michael Tailor, AI Ethics Researcher
10. Case Study: An Ethical AI Success Story
Bank X, a leading financial institution, recently incorporated an AI-driven investment tool. However, instead of blindly adopting the technology, they partnered with a B2B SaaS provider specializing in ethical AI solutions. This collaboration ensured:
- A thorough audit of data sources to eliminate biases.
- The implementation of strong encryption measures to safeguard data.
- Transparent reporting allowing clients to understand AI-driven decisions.
This proactive method not best superior patron agree with however also set Bank X as an industry benchmark for moral AI practices.
11. FAQ
Q1. What is ethical AI in the context of financial services?
A1. Ethical AI refers to the accountable layout, development, and deployment of artificial intelligence in a way that upholds ethical and moral requirements. In economic services, it method the usage of AI tools and solutions which can be transparent, honest, unbiased, and respectful of customers' rights, making sure that financial selections made by means of AI benefit anybody and do no harm.
Q2. Why is ethics important in financial AI systems?
A2. Trust is the cornerstone of the economic quarter. Ethical AI enables build and maintain this trust. It ensures that AI-pushed choices are transparent, truthful, and devoid of biases, promoting an equitable monetary environment for all stakeholders.
Q3. How can we ensure AI models in finance are free from biases?
A3. Ensuring independent AI fashions requires diverse schooling facts, non-stop tracking of AI decisions, and recurring reviews for any signs of biases. Open channels for comments and the usage of external audits can also provide insights into ability biases.
Q4. What role does the feedback loop play in ethical AI?
A4. A remarks loop is essential for non-stop improvement. It involves spotting mistakes, analyzing them, making important adjustments, after which speaking these changes. This iterative system allows refine AI fashions, ensuring they continue to be moral through the years.
Q5. Are there regulations in place for ethical AI in finance?
A5. While specific regulations vary by country, there's a growing focus on developing standards and guidelines for AI in finance. Many institutions also adhere to internal ethical guidelines and collaborate with B2B SaaS providers to ensure compliance with best practices.
Q6. Can ethical AI be a competitive advantage for financial institutions?
A6. Absolutely! In a sector where trust is paramount, showcasing a commitment to ethical AI can enhance brand reputation, foster customer loyalty, and even open doors to new market segments concerned about ethical tech usage.
Q7. How can financial institutions stay updated on ethical AI trends and best practices?
A7. Staying updated requires a mix of continuous learning, collaboration with experts, participation in industry forums, and engagement with B2B SaaS providers specializing in ethical AI solutions.
Q8. Is there a trade-off between AI performance and ethics?
A8. Not necessarily. While some argue that stringent ethical practices might limit AI's potential, many believe that in the long run, ethical AI leads to more sustainable and trustworthy solutions, which is beneficial for both institutions and their customers.
Q9. How do we ensure transparency in complex AI algorithms?
A9. Ensuring transparency can be hard, in particular with deep studying models. However, strategies like explainable AI (XAI) are emerging to make even complicated models more comprehensible. Additionally, clear documentation, open communique channels, and periodic opinions can in addition enhance transparency.
Q10. What's the future of ethical AI in finance?
A10. As AI will become extra intertwined with monetary offerings, ethical considerations will best develop in importance. The future probable holds tighter regulations, greater state-of-the-art moral AI tools, and a stronger emphasis on transparency, equity, and collaboration.
12. Conclusion: The Road Ahead for Ethical AI
The journey of AI in financial services is simply starting, and the moral considerations are evolving alongside it. By embracing records protection, taking part with B2B SaaS providers, and continuously studying from actual-global applications, the monetary enterprise can navigate this moral panorama efficaciously.
Remember, at the heart of every AI-pushed choice have to be the human touch, ensuring equity, transparency, and recognize for all.
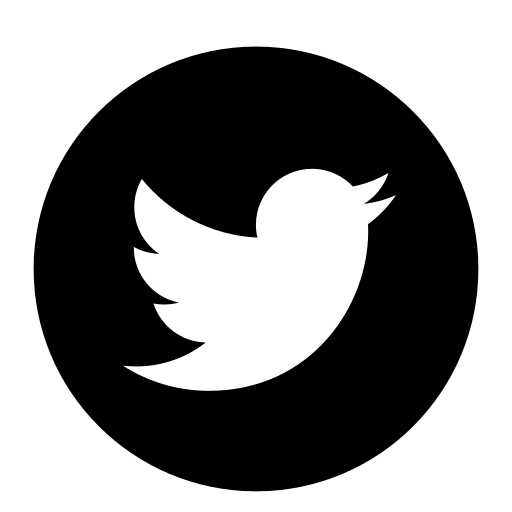
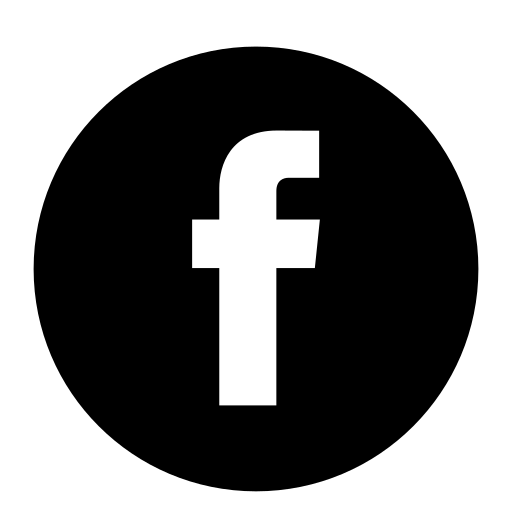
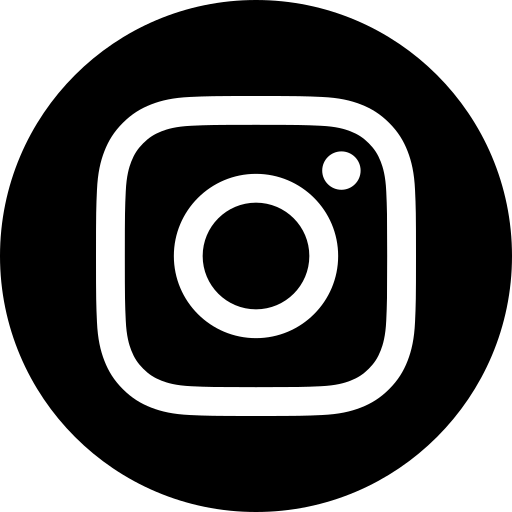