Driving Innovation: How AI Agents are Fueling Progress in the Pharmaceutical Sector

1. Introduction: The Rise of AI in Pharma
The integration of synthetic intelligence (AI) into the pharmaceutical industry indicates a transformative moment, a paradigm shift that could be in comparison to the arrival of biotechnology or the discovery of the double helix structure of DNA. Let's delve deeper into the various facets of this integration:
1. Historical Context
Historically, drug discovery and development were often processes that relied heavily on trial and error, which were both time-consuming and costly. However, with the advent of computational tools in the late 20th century, things began to change. But it is handiest with the latest upward thrust of AI and gadget getting to know that we have all started to unencumber honestly transformative potential. This isn't simply another tech fashion; this is a progressive method that may redefine healthcare and remedy as we comprehend it.
2. AI-Driven Drug Discovery
AI has the capability to analyze vast and complex datasets, something that would take humans years, in mere minutes. This capability can:
- Identify potential drug candidates from vast molecular databases.
- Predict how different drugs can interact with various biological systems, reducing adverse reactions.
- Tailor treatment strategies for individual patients based on their genetic makeup.
In simple terms, what used to be a shot in the dark is now becoming a more precise, guided missile in targeting diseases.
3. Clinical Trial Optimization
Clinical trials are a pivotal and expensive phase in drug development. AI can:
- Predict which patients are most likely to benefit from a trial.
- Monitor patients in real-time and adjust treatment protocols accordingly.
- Analyze results rapidly, accelerating the feedback loop and potentially getting vital drugs to market faster.
4. Personalized Medicine
With the integration of AI, we're moving from a 'one-size-fits-all' approach to a more individualized form of treatment. By reading someone's genetic facts, AI can predict how they could react to sure pills or remedies, paving the way for treatments tailor-made to man or woman wishes.
5. Addressing Challenges
Of course, this AI-driven transformation doesn't come without its challenges:
- Data Privacy: With the processing of vast amounts of patient data, ensuring data privacy and ethical use is crucial.
- Technical Hurdles: The integration of AI tools with existing pharmaceutical processes can be complex.
- Regulatory and Trust Issues: Ensuring that AI-driven processes meet regulatory standards and gain the trust of both professionals and the public is paramount.
2. AI and Information Security: The Perfect Marriage
In today's hyper-connected era, information security (or InfoSec) is at the forefront of organizational priorities. With increasing amounts of data being stored, transmitted, and processed digitally, the threat landscape is expanding at an alarming rate. Enter Artificial Intelligence (AI). The convergence of AI with InfoSec is reshaping the way organizations defend against threats, ensuring a proactive, adaptive, and resilient approach. Let's delve deeper into this union:
1. Dynamic Threat Detection
Traditional security systems operate based on predefined rules, making them less effective against novel or evolving threats. AI-driven security solutions, on the other hand:
- Learn from historical data to recognize patterns associated with malicious activities.
- Continuously adapt and evolve to recognize new threat vectors, ensuring that defenses are not just static but are ever-evolving.
- Offer a level of real-time responsiveness that rule-based systems can't match.
2. Predictive Analysis for Proactive Defense
Instead of merely reacting to security breaches, AI empowers organizations to anticipate them:
- By analyzing vast datasets, AI can identify subtle anomalies that might indicate a potential future attack.
- This "early warning system" allows IT teams to address vulnerabilities before they're exploited.
3. Automated Incident Responses
When a breach occurs, time is of the essence. AI-enhanced systems:
- Can automatically isolate affected systems to prevent further spread of the breach.
- Initiate predefined security protocols, often quicker than a human responder could, minimizing potential damage.
4. Phishing Attack Mitigation
One of the most common cyber threats today is phishing. AI assists in:
- Analyzing email patterns and content to detect unusual or suspicious activities.
- Offering real-time warnings to users and administrators about potential phishing attempts.
5. Enhancing User Authentication
Passwords alone are no longer considered secure. AI supports multi-factor authentication (MFA) by:
- Analyzing behavioral patterns, such as keystroke dynamics or mouse movements, to authenticate users with greater accuracy.
- Offering adaptive authentication, where the type of verification might change based on the risk associated with a user's current behavior.
6. Insider Threat Detection
Not all threats come from outside an organization. Detecting insider threats is particularly challenging:
- AI systems can monitor user activities and compare them against baselines.
- Unusual data access patterns or file transfers can be flagged, ensuring that potential insider threats are swiftly identified.
3.How SaaS is Powering AI-Driven Pharmaceuticals
In state-of-the-art digital era, the interplay among Software-as-a-Service (SaaS) and synthetic intelligence (AI) is revolutionizing diverse industries. One of the most profound influences may be seen within the pharmaceutical region, where SaaS structures are supercharging the competencies of AI-pushed drug development and research.
1. Seamless Data Management
With the vast amounts of data generated in pharmaceutical research, there's a dire need for seamless data management. Traditional systems often struggled with scalability, speed, and efficiency. SaaS solutions, on the other hand:
- Offer cloud-based storage, ensuring scalable data infrastructure.
- Facilitate real-time access to data from any location, fostering collaboration.
- Ensure automatic backups and data redundancy, which are critical in research environments.
2. Advanced Analytical Capabilities
SaaS platforms are equipped with state-of-the-art analytical tools tailored for the pharmaceutical industry:
- Predictive Analysis: Anticipate drug responses based on historical data, ensuring safer and more efficient clinical trials.
- Data Visualization: Transform complex drug development data into visual formats for easier comprehension and decision-making.
- Real-time Monitoring: Monitor ongoing trials, patient responses, and other critical metrics in real time.
3. Facilitating Collaboration
Gone are the days when researchers had to work in silos. SaaS platforms promote collaboration by:
- Allowing multiple researchers to work on the same dataset simultaneously.
- Offering communication tools integrated directly into the platform.
- Facilitating the sharing of insights, data, and methodologies globally.
4. Scalable Computational Resources
AI-driven drug discovery requires significant computational power. SaaS platforms offer:
- Access to cloud-based computational resources.
- The ability to scale up or down based on project requirements.
- Cost savings, as organizations pay only for the resources they use.
5. Enhanced Security and Compliance
In an industry where data security and regulatory compliance are paramount, SaaS platforms shine by:
- Implementing advanced encryption techniques to protect sensitive data.
- Automating compliance workflows to ensure adherence to global regulations.
- Regularly updating to address the latest security threats and compliance requirements.
6. Plug-and-Play Integration
For pharmaceutical companies venturing into AI-driven research, SaaS platforms offer:
- Easy integration of AI modules, eliminating the need for in-house AI expertise.
- Compatibility with a wide range of AI tools and algorithms.
- Opportunities for customizing AI models based on specific research requirements.
4. Embracing B2B Software for Advanced Drug Development
In the intricate dance of drug development, where time is of the essence and the stakes are high, businesses are increasingly seeking tools that can streamline processes, enhance collaboration, and reduce time-to-market. Business-to-Business (B2B) software program solutions have emerged as those indispensable tools, proving transformative for the pharmaceutical global.
The Rationale Behind Adopting B2B Software
- Data-Driven Decision Making: In the age of Big Data, pharmaceutical companies are drowning in vast amounts of research data. B2B software program program facilitates shrewd information processing, enabling corporations to extract actionable insights, be it understanding patient responses, gauging drug interactions, or predicting market call for.
- Integrated Workflows: With numerous departments and cross-functional teams often working in silos, there's an inherent need for a unified system. B2B software answers provide incorporated platforms that foster seamless conversation, making sure that everyone is on the identical web page, from studies and development to advertising and sales.
- Global Collaboration: Given the international nature of pharmaceutical giants, fostering real-time collaboration across continents is paramount. Cloud-based B2B platforms offer this, ensuring that scientists, regardless of location, can work cohesively on shared projects.
Examples of B2B Software in Pharma
- Clinical Trial Management Systems (CTMS): Such platforms assist in planning, tracking, and managing clinical trials, ensuring timely patient recruitment, adherence to regulatory requirements, and efficient data capture.
- Pharmacovigilance Systems: These gear resource in shooting, reporting, and analyzing detrimental drug reactions, crucial for patient safety and regulatory compliance.
- Supply Chain Management Platforms: In the pharmaceutical world, managing the drug supply chain is intricate. B2B software helps in optimizing logistics, ensuring timely drug deliveries, and reducing wastage.
Challenges & Solutions
While the adoption of B2B software is beneficial, it's not without its challenges:
- Integration with Legacy Systems: Many pharmaceutical companies still rely on outdated systems. However, several B2B software providers now offer integration tools, making the transition smoother.
- Cost Implications: Upfront investment in B2B solutions can be daunting. Yet, when considering the long-term benefits, such as reduced R&D timelines and efficient resource utilization, the ROI becomes evident.
- Training & Adoption: Introducing a new system can face resistance from employees accustomed to old methods. By supplying complete education and demonstrating the tangible benefits of those structures, organizations can ensure a smoother adoption curve.
5. Quotes to Ponder
1. On AI in Pharma:
"In the intricate dance of science, AI is the choreographer guiding pharmaceuticals in a rhythm of precision and innovation."
2. Regarding Information Security and AI:
"Just as a vault safeguards treasures, AI stands sentinel, ensuring every byte of pharmaceutical data remains untouched and secure."
3. The Impact of SaaS on AI-Driven Pharma:
"SaaS and pharmaceuticals: a modern-day symbiosis where digital clouds propel groundbreaking discoveries on the ground."
4. The Influence of B2B Software in Drug Development:
"B2B software in pharma is like a compass in uncharted waters – guiding, enabling, and fostering innovation at every turn."
5. Machine Learning's Role in Predictive Analysis:
"Like a soothsayer predicting the future, machine learning delves deep into data, illuminating the path of drug discovery."
6. AI's Contribution to Regulatory Compliance:
"In the intricate labyrinth of regulations, AI emerges as the beacon, ensuring every step taken in pharma is compliant and sure."
7. B2B SaaS as the Facilitator for AI Integration:
"As bridges connect lands, B2B SaaS serves as the bridge connecting the vast worlds of AI and pharma, fostering seamless integration and boundless innovation."
8. On the Challenges and Opportunities Ahead:
"While the confluence of AI and pharma is not without its rapids, it's the very turbulence that carves out opportunities for those daring to navigate."
6 . Leveraging Machine Learning for Predictive Analysis in Drug Discovery
The intersection of system mastering (ML) and drug discovery is undeniably one of the maximum transformative collaborations in modern technological know-how. By harnessing the electricity of ML algorithms, researchers can sift via sizeable datasets, decipher styles, and make predictions that could be insurmountably time-ingesting for humans to make. Let's delve deeper into how ML is reshaping drug discovery:
1. The Rise of Personalized Medicine
The one-size-fits-all model of medication is becoming increasingly obsolete. With the integration of ML in pharmacogenomics – the study of how genes influence a person's response to drugs – there's a surge in tailoring drug treatments to individual genetic makeups.
- Patient Data Analysis: ML algorithms can analyze a patient's genetic data to predict how they might respond to certain drugs, paving the way for treatments specifically tailored to each individual.
- Adverse Reaction Prevention: By understanding an individual's genetic makeup, ML can also predict potential adverse reactions, ensuring safer drug prescriptions.
2. Deciphering Drug Interactions
One of the most significant challenges in drug discovery is predicting how a new drug will interact with other medications and biological systems.
- Drug-Drug Interaction: Machine learning models are being developed to predict harmful interactions between different drugs, even before they manifest in clinical trials.
- Metabolic Pathway Analysis: ML can simulate and examine complex metabolic pathways, supporting researchers understand how a drug might be metabolized and what byproducts is probably produced.
3. Optimizing Clinical Trials
Clinical trials are both time-consuming and expensive. By integrating machine learning, pharmaceutical companies can optimize these trials in various ways.
- Candidate Selection: ML can sift through vast datasets to identify the most promising drug candidates, ensuring resources are utilized most effectively.
- Patient Recruitment: Machine studying can examine patient statistics to identify perfect applicants for clinical trials, main to more correct effects and quicker drug approvals.
- Real-time Data Analysis: With ML, real-time data from clinical trials can be analyzed, allowing for rapid adjustments and decision-making.
4. Drug Repurposing
It's not always about discovering new drugs; sometimes, it's about finding new uses for old ones. Machine learning has shown potential in identifying such opportunities.
- Pattern Recognition: By analyzing large datasets, ML can identify patterns suggesting that a drug, initially developed for one condition, might be effective for another.
- Cost Reduction: Drug repurposing, aided by ML, can significantly reduce the cost of bringing a drug to the market, as the drug has already undergone numerous safety tests.
5. AI-driven Drug Design
Beyond just analysis, machine learning is now venturing into the realm of drug design.
- Molecular Simulations: ML algorithms can simulate how different molecular structures will behave, enabling the design of new drugs from the ground up.
- Predictive Analysis: With ML's predictive capabilities, researchers can forecast how a proposed drug might interact with biological systems, fine-tuning its design before any synthesis occurs.
7. AI in Streamlining Regulatory Compliance
The pharmaceutical sector, revered for its step forward discoveries, is likewise one of the most closely regulated industries. Ensuring pills are both effective and secure for public intake is paramount, and as such, companies often find themselves navigating a labyrinth of regulatory necessities. In this complex ecosystem, Artificial Intelligence (AI) has emerged as a beacon, providing solutions that no longer most effective streamline compliance techniques but additionally redefine them.
1. Automated Data Audits
Historically, audits of clinical trials, research data, and production processes were primarily manual endeavors, consuming time and being prone to human error.
AI-powered Audits:
- Speed: Machine learning models can scan vast datasets in a fraction of the time a human would require.
- Accuracy: AI algorithms, once trained, exhibit consistent performance, eliminating the inconsistencies of human audits.
- Continuous Monitoring: Rather than periodic checks, AI systems can provide real-time, ongoing audits, ensuring constant compliance.
2. Real-time Reporting
In the face of a potential compliance issue, time is often of the essence. Delays can result in financial penalties or, worse, risks to patient safety.
AI's Role in Reporting:
- Instant Data Retrieval: Natural Language Processing (NLP) tools can instantly extract relevant information from vast datasets.
- Predictive Analysis: AI can forecast potential compliance breaches, allowing firms to be proactive rather than reactive.
- Visualization: Advanced AI tools generate intuitive visual reports, aiding in swift decision-making processes.
3. Reducing Human Error
Human error, whether unintentional or due to oversight, has historically been a significant challenge in regulatory compliance.
How AI Mitigates This:
- Standardization: AI tools ensure a standardized process, minimizing variability.
- Learning from Mistakes: Machine learning models can learn from previous errors, refining their processes over time.
- Assistance and Oversight: AI doesn't necessarily replace humans but augments their capabilities, offering guidance and double-checking manual inputs.
4. Scalable Compliance for Global Operations
Pharmaceutical companies often operate on a global scale, meaning they must adhere to varying regulatory standards across different regions.
AI's Global Approach:
- Customized Algorithms: AI models can be tailored for specific regional regulations, ensuring localized compliance.
- Multilingual Processing: Using NLP, AI tools can process and audit documents in multiple languages, ensuring global standardization.
- Cross-border Data Synthesis: AI can consolidate data from various regions, ensuring a unified compliance strategy.
5. Feedback Loops and Continuous Improvement
With AI, it's not just about maintaining the status quo; it's about continuous enhancement.
AI-driven Feedback Mechanisms:
- Adaptive Learning: As regulations evolve, machine learning models can adapt, ensuring they're always up-to-date.
- Feedback Integration: AI systems can integrate feedback from regulatory bodies, refining their processes in real-time.
- Benchmarking: AI tools can compare a company's compliance processes with industry standards, highlighting areas for improvement.
8. The Role of B2B SaaS in Facilitating AI Integration
In today's dynamic digital landscape, B2B (Business-to-Business) Software as a Service (SaaS) platforms play a pivotal role in the widespread integration and adoption of Artificial Intelligence (AI). The seamless fusion of SaaS with AI technologies has catapulted companies, particularly the ones within the pharmaceutical place, into a latest age of innovation, optimization, and productivity. Here's a deep dive into the characteristic and importance of B2B SaaS in this transformative journey.
1. Seamless Integration of AI Capabilities
B2B SaaS platforms often come equipped with ready-to-use AI modules, eliminating the need for companies to develop AI algorithms from scratch. This plug-and-play model:
- Reduces Development Time: Companies can quickly leverage AI's power without undergoing lengthy development cycles.
- Ensures State-of-the-Art Tools: SaaS providers constantly update their AI tools, ensuring businesses have access to the latest and most efficient algorithms.
- Minimizes Initial Costs: Instead of hefty upfront investments in AI infrastructure, companies can utilize SaaS subscription models to access top-tier AI capabilities.
2. Cloud-Driven Computational Power
One of the major challenges in AI integration is the vast computational power required, especially for complex tasks like deep learning. B2B SaaS platforms, being cloud-based, provide:
- Scalable Resources: Companies can scale their computational needs based on the task, without investing in physical infrastructure.
- Data Storage and Management: The cloud infrastructure ensures data is stored securely and can be accessed and processed rapidly.
- Collaborative Environments: With data on the cloud, teams can collaborate in real-time, regardless of their geographical location.
3. Democratizing AI Access
Not every company has the resources or expertise to develop AI tools in-house. B2B SaaS platforms democratize access to AI by:
- Offering Intuitive Interfaces: Even those without a deep understanding of AI can harness its capabilities through user-friendly SaaS interfaces.
- Providing Training and Support: Most SaaS platforms offer extensive training and support, ensuring companies can maximize the benefits of AI integration.
- Facilitating Customization: Companies can tailor SaaS AI tools to their unique needs, ensuring relevancy and efficiency.
4. Ensuring Regulatory and Ethical Compliance
AI, especially in sensitive sectors like pharmaceuticals, comes with a host of regulatory and ethical concerns. B2B SaaS platforms can:
- Automate Compliance Checks: AI algorithms can be pre-configured to adhere to industry-specific regulations.
- Provide Transparent AI Models: To address the 'black box' nature of some AI models, SaaS platforms can offer tools that make AI decisions more transparent and understandable.
- Regularly Update Algorithms: As regulations evolve, SaaS providers can update their tools to ensure continued compliance.
9 . The Road Ahead: Challenges and Opportunities
The journey of integrating AI into the pharmaceutical industry, even as laden with monstrous capacity, isn't with out its twists and turns. As agencies forge ahead, they may be met with a complex tapestry of challenges that require revolutionary solutions. Let's delve deeper into those demanding situations and the corresponding possibilities they present.
1. Data Privacy Concerns
In an age where data breaches are all too common, the pharmaceutical sector, which handles sensitive patient data, cannot afford to be lax.
Challenges:
- Protecting patient identities while harnessing data for research.
- Navigating global data protection regulations which may vary by region.
- Ensuring third-party vendors adhere to stringent data privacy norms.
Opportunities:
- Development of advanced encryption techniques tailored for healthcare data.
- Collaborative global efforts to standardize data protection norms.
- Offering specialized training programs to raise awareness about data privacy among staff.
2. Interpretable AI Models
AI and machine learning models, particularly deep learning, are often criticized for being 'black boxes', where the decision-making process is obscured.
Challenges:
- Researchers and doctors might be hesitant to trust predictions without understanding the underlying reasoning.
- Regulatory bodies might require explanations for AI decisions, especially in critical areas like patient care.
Opportunities:
- Advancement in the field of explainable AI, which seeks to make AI decisions more transparent.
- Collaborative research initiatives aimed at building trust in AI models.
- Developing frameworks for rigorous validation and verification of AI systems in healthcare.
3. Integration Hurdles
Merging the traditional and often rigid processes of the pharmaceutical industry with the dynamic nature of AI tools can be akin to fitting a square peg in a round hole.
Challenges:
- Legacy systems in pharmaceutical companies might not be readily compatible with new AI solutions.
- Resistance to change within organizations, given the high stakes in the pharmaceutical sector.
- High initial costs and perceived risks associated with AI integration.
Opportunities:
- B2B SaaS companies can develop bespoke solutions tailored for seamless integration with legacy systems.
- Organizational change management initiatives to foster a culture of innovation and adaptability.
- Demonstrating the long-term ROI of AI integration through pilot projects and case studies.
10. FAQ:
Q1: How is AI revolutionizing the pharmaceutical sector?
A1: AI is streamlining drug discovery, personalizing treatments, optimizing clinical trials, and enhancing data security within the pharmaceutical industry.
Q2: Why is information safety vital within the pharma area?
A2: Given the touchy nature of patient information and proprietary studies, it's critical to ensure this statistics remains confidential and protected from capacity breaches.
Q3: What blessings do SaaS solutions provide to pharmaceutical businesses?
A3: SaaS systems offer scalability, cost-effectiveness, interoperability, and easy integration of AI tools, making them ideal for the evolving needs of the pharmaceutical quarter.
Q4: How are B2B software solutions enhancing drug development?
A4: B2B software facilitates real-time collaboration, intuitive data visualization, and ensures regulatory compliance, thus streamlining and enhancing the drug development process.
Q5: Can AI models predict potential harmful drug interactions?
A5: Yes, with the vast amounts of data available, AI models can analyze and predict possible harmful interactions between drugs before they reach the market.
Q6: Are there challenges in integrating AI within the pharmaceutical industry?
A6: While AI offers numerous benefits, challenges such as data privacy concerns, ensuring AI model interpretability, and integration hurdles with traditional processes persist.
Q7: How does B2B SaaS facilitate AI integration in pharma?
A7: B2B SaaS platforms often offer plug-and-play AI modules, cloud-based AI training resources, and collaborative research tools, making AI integration more seamless for pharmaceutical companies.
Q8: Is patient privacy at risk with the adoption of AI in pharma?
A8: While AI models require large amounts of data, robust encryption and anonymization techniques are employed to ensure patient data remains confidential and secure.
Q9: How can pharmaceutical companies ensure AI models are not just 'black boxes'?
A9: By investing in explainable AI (XAI) tools and methodologies, companies can ensure that AI models are interpretable and their decisions can be understood by researchers.
Q10: Are there B2B SaaS solutions tailored specifically for the pharmaceutical industry?
A10: Absolutely. Many SaaS providers are now offering solutions specifically designed to meet the unique needs and challenges of the pharmaceutical sector.
11. Conclusion: The Future of Pharma with AI
The integration of AI sellers within the pharmaceutical quarter indicators a new sunrise. As the realms of AI, information security, SaaS, and B2B software converge, we stand at the cusp of a revolution. For those in the B2B SaaS industry, the road ahead is clear: innovate, integrate, and lead the charge.
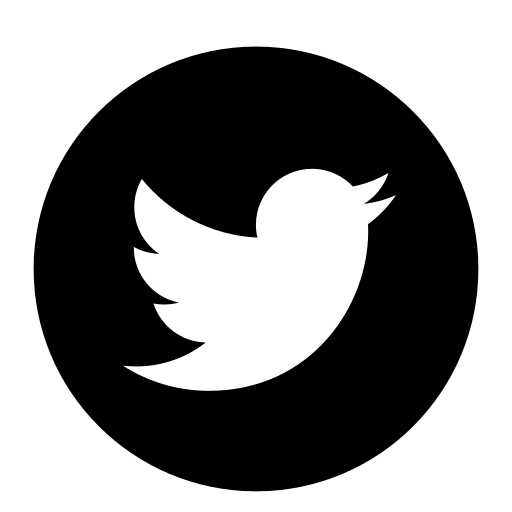
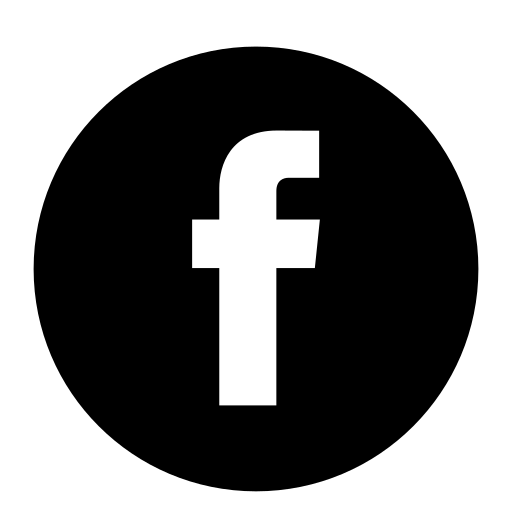
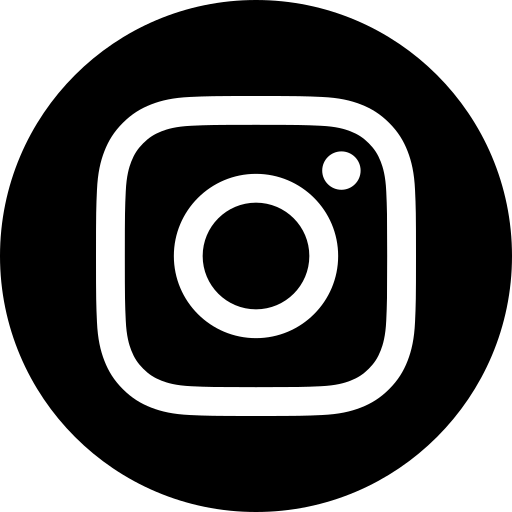